SDMS redefined: Making sure you have a solution, and not a swamp, for your scientific data
Scientific data management is more important than ever before. We walk through three key features to look for in any modern SDMS.
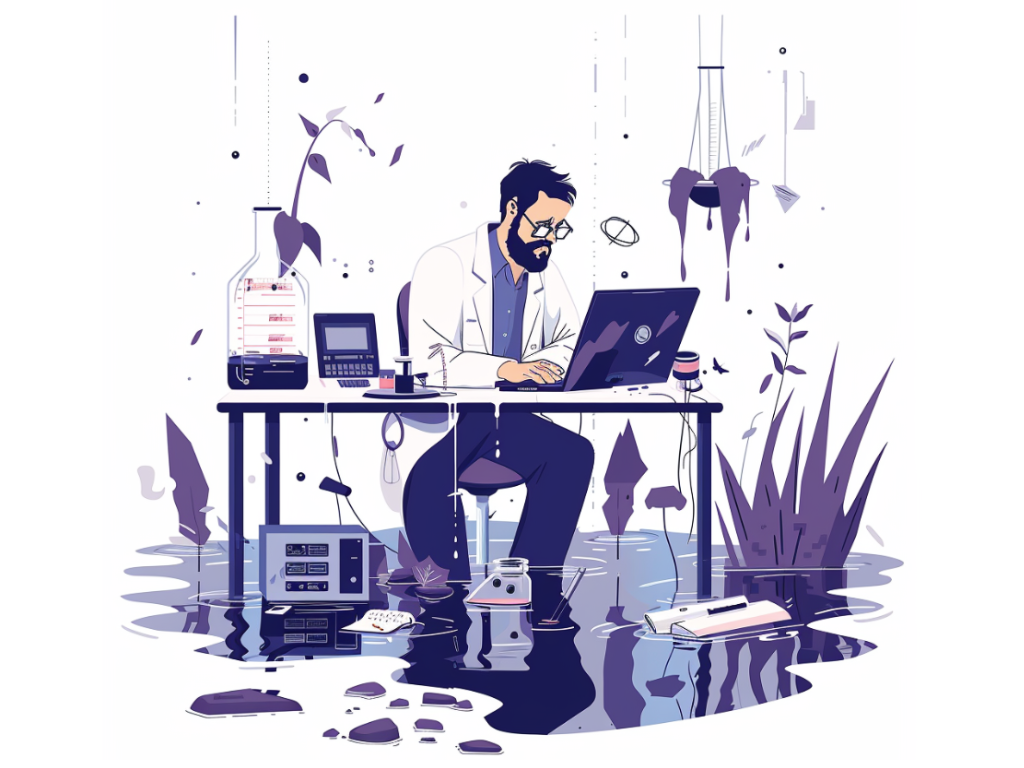
Does “scientific data management system” sound intimidating? You’re not alone. The phrase might conjure images of expensive consulting contracts, or a big six-figure scientific tech purchase. Maybe it even brings up bad memories of subpar scientific data management system (SDMS) software.
But scientific data management and SDMS platforms have come a long way over the last decade. Scientific data management has never been more important, and SDMS platforms are ripe for redefinition—as are the data they store. With the rise of automation, AL/ML, and other advanced technologies, biotech organizations have realized that the raw data that lives within an SDMS is just as important as finished data. In fact, it might hold untapped insights, discoveries, and opportunities that labs would otherwise miss.
In this post, we’re exploring:
- Why scientific data management is crucial for biotechs today
- Why SDMS platforms should be part of the biotech tech stack
- Three key features to look for in a modern, cloud-native SDMS
Why SDMS Platforms Have Been Sidelined
Scientific data is complex—that’s one thing anyone in biotech can agree on. It’s especially true in R&D and the wet lab, where experiments resemble custom craftsmanship more than the large-scale assembly line of commercialized products. Wet lab data comes from lots of different sources and instruments, is iterative, and is customized from experiment to experiment.
Over the years, labs have tried to find a good way to manage and trace all that complex, mismatched data. SDMS solutions emerged to answer that need. But, so far, they’ve been a sleepy part of the biotech tech stack. Faced with so much heterogenous data, SDMS tools have primarily been used as storage–nay, a dumping ground–for data. As a result, that data is typically dormant and mostly unusable.
In addition, many SDMS solutions have historically had limited functionality:
- Sporadic or one-off integrations with other parts of the tech stack, such as LIMS tools
- Narrow ability to perform data analysis
- “Add on” design, bundled with a bigger tech solution or software
Most SDMS solutions on the market today have been a dead end for lab data. Many labs have even turned to non-biotech app tools like Sharepoint, G-Drive, Dropbox. But these aren't ideal either, since none are designed specifically for lab data. They lack crucial features, such as the ability to track metadata around experiment runs. The alternative is building everything in-house from scratch using a raw cloud provider like AWS. Naturally, that comes with its own set of difficulties and costs that we've discussed in depth before.
All too often, labs don’t even know what they’re missing with a non-specialty storage solution. But as data becomes more heterogenous than ever, it's time for biotech organizations to rethink what an SDMS platform can do for their lab.
Three Key Features Of A More Dynamic SDMS
Many biotech startups approach scientific data management the same way: "let's just shove it all into a repository." But over time, this approach starts to cause problems: lost data, poor context, and missed opportunities.
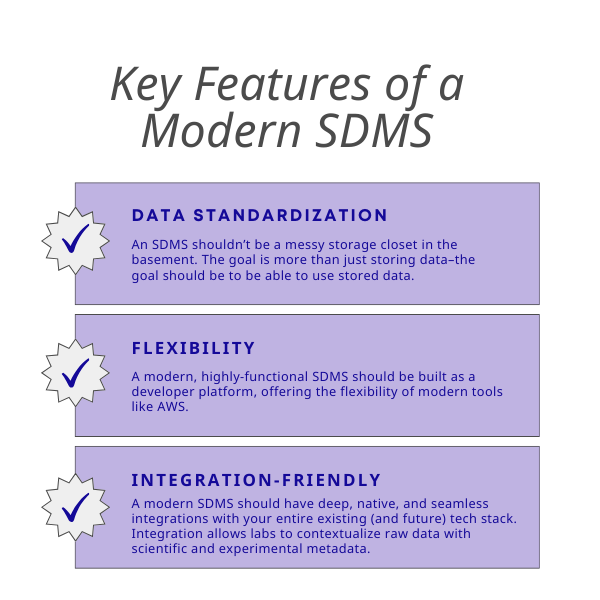
But a dynamic SDMS solution can offer more than storage, bringing rich structure to data, empowering advanced analysis, integrating data across the organization, and more.
When adding an SDMS platform to your biotech tech stack, look for these three key pieces of functionality:
1. Data Standardization
For many biotechs, data is stored higgledy-piggledy across different pieces of software, physical notebooks, and Excel files. An SDMS shouldn’t be a messy storage closet in the basement. The goal should be more than just storing data.
Yes, an effective SDMS should be powerful enough to ingest data from a wide variety of instruments. But more importantly, it must have the capability to organize that disparate data and prepare it for further application and use cases. In short, any good modern SDMS platform should enable labs to make their data meet FAIR principles:
- Findable across the organization and all experiments
- Accessible to anyone who needs it, when their need it
- Interoperable, so scientists can bring together datasets from different sources easily, including instruments, lab equipment, external partners like CMDOs and more
- Reusable across different analyses, empowering scientists to cross-compare data and uncover new insights and discoveries
Plus, with standardization, labs will be able to do more with their data and lay a strong foundation for advanced tech tools, like automation and AI/ML.
2. Flexibility
Many legacy solutions are clunky, offer few customizations, require complicated implementations, and have many limitations when it comes to their capabilities. For example, an SDMS specific to chromatography may only be set up to analyze that specific data type. Unfortunately, that chromatography data set is likely just one piece of many data points in the overall workflow for those samples.
A modern, highly-functional SDMS should be built as a developer platform, offering the flexibility of modern raw cloud tools like AWS. First and foremost, implementation must be easy and intuitive for even the amateur users. At the same time, more seasoned users should be able to modify and customize it to better suit their complex needs and evolving workflows.
Beyond deployment, a modern SDMS should standardize raw data according to whatever ontology an organization has chosen, such as Allotrope. Cloud-compatibility goes without say, though being cloud-native is ideal.
3. Tons of Integration-Friendly Features
Historical SDMS tools haven’t been well-integrated. Today, almost every biotech has a toe in the cloud via their ELN solution, even if they’re not too far down the lab digitalization maturity curve. That’s why any SDMS worth considering today will be compatible with any tool or piece of software that touches lab data.
A modern SDMS should have deep, native, and seamless integrations with your entire existing and future tech stack, including:
- Systems of record, like a LIMS tool
- Workflows, such as bioinformatics or ML
- Fit-for-purpose logic
- Analysis tools for primary and secondary interpretation of the data
- And more (see our "Four Corners" post for a more extensive list of frontends and backends that should integrate with an SDMS)
By integrating with the entire tech stack, a modern SDMS enables labs to contextualize raw data with scientific and experimental metadata. It also allows scientists to better slice and dice experimental results to uncover hidden insights and discoveries. In science, you don't know what you don't know. The job of a good SDMS tool is to help reveal something unexpected.
Scientific Data Management Is More Than Just an App
SDMS platforms shouldn't just be an afterthought, or just another app. Today, there’s a need for a scientific data management platform that actually manages data, organizes it, and makes it actionable for further work. It’s time to change the mindset around SDMS platforms from being data swamps to actual solutions.
A modern, biotech-specific, cloud-based SDMS is an essential and foundational part of any biotech’s data infrastructure. It easily connects and integrates with the rest of the lab’s hardware and software tech stack, creating continuity and eliminating confusion.
Banish any scary associations from past SDMS fails and embrace the new generation of data management in biotech—your data will thank you.