Structure and Flexibility: Two Strategies to Take Control of Your Scientific Data Management
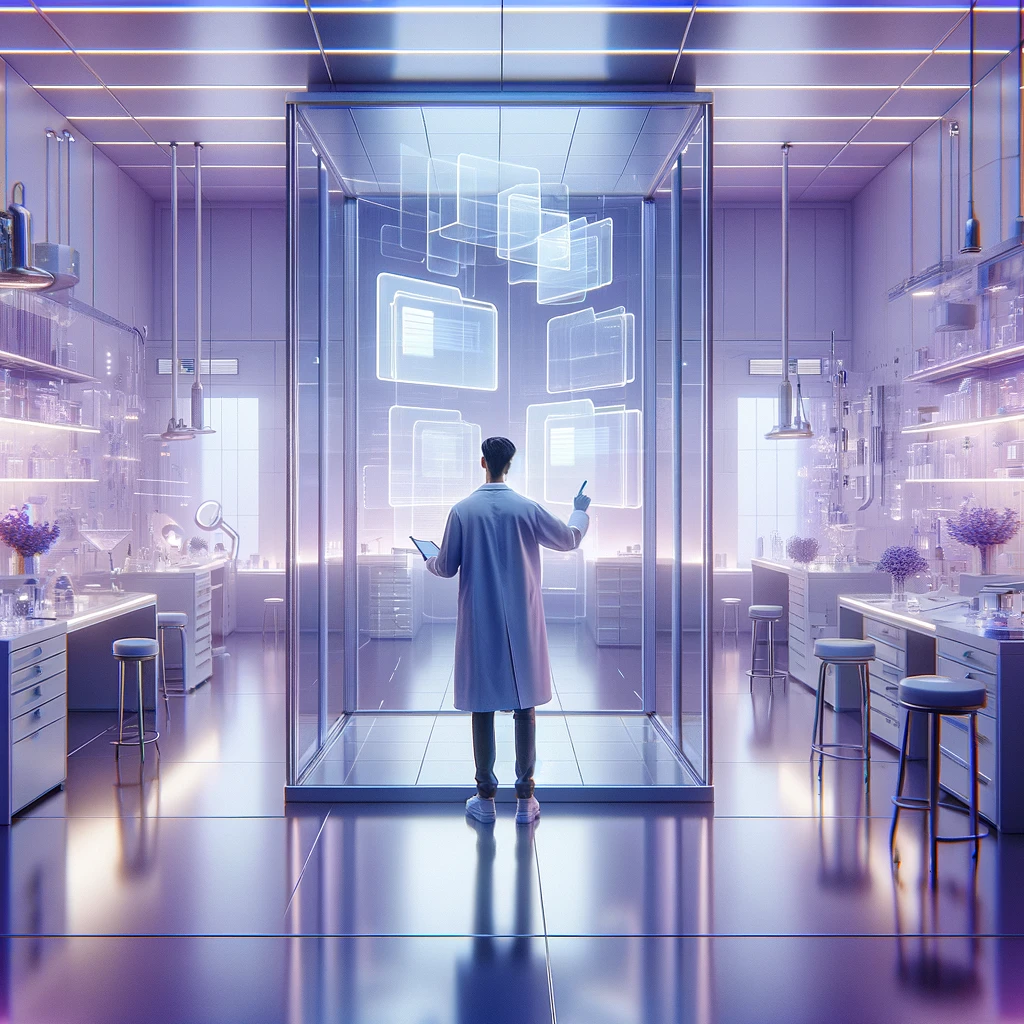
ELN. LIMS. QMS. When it comes to lab data, there are a lot of three to four-letter acronyms. For many people, an ELN, shorthand for an electronic lab notebook, is arguably the most important. In fact, at Ganymede, many of the biotech companies we talk with view their ELN as the foundation of all their data management, from collection to analysis. Everything goes into it.
However, there’s something just as important as an ELN in a lab’s tech stack: structured file storage that flexibly captures metadata. When labs rely solely on ELNs to store everything in an unstructured format, they run into challenges. For example, lab data needs to be FAIR: findable, accessible, interoperable, and reusable. But when data is stored in an ELN, it’s not associated with other parts of a bigger experiment, and files often end up in Sharepoint or in haphazard locations. ELNs require a complementary storage solution.
We think there is a better way to store scientific data reliably for the long term with data visibility: a scientific data management system (SDMS). With a biotech-specific, cloud-native SDMS to complement their ELN, labs can meet FAIR principles, associate all the necessary information across instruments and non-instrument data points, and support complex downstream analyses.
While it may seem like a niche term, an SDMS has become increasingly essential to growing labs in the last decade. Today, a great SDMS supports lab automation, stores files in a structured way, and makes data FAIR.
Ganymede offers a modern cloud-native SDMS. With our SDMS, labs can:
- Automatically capture files from lab instruments
- Store and easily retrieve files
- Push data automatically to their LIMS
- Generate audit logs and support general compliance efforts
- Parse files and tables
- And more.
There are several key principles to effectively managing your scientific data. Below, we walk through two ideas and the way Ganymede’s cloud-native SDMS platform can support them.
Structure your data strategically
Companies often take a catch-all approach to storing data: they grab instrument data and shove it into an ELN. While the instinct there is correct—we always advocate for saving everything—the methodology is usually scattershot. ELNs typically store unstructured data. Without a broader data strategy or established structure, people file things and quickly forget about them.
The result? A giant data swamp full of information that’s hard to find, hard to understand, and hard to use.
Companies can take control of their scientific data with a more strategic approach to data collection and organization. An ELN should not just be an alternative to pen/paper—it’s a valuable port for tracking inputs/outputs from experiments (part of what we call the four corners of digitalization).
Context is key. Start with understanding your goals: what you want to capture, where the data is coming from, and where it’s going. This forms a backbone of metadata that you should capture for every file.
Some important questions to ask include:
- What context do we want to capture about the data in our experiments?
- What experiments and assays are we conducting?
- What kinds of files are we generating, and what are the next steps?
- How will we name the files?
That last point is especially relevant: come up with a naming convention and a template. Use something clean and easy to read. Automate data collection, so that scientists can focus on other tasks. By ingesting data directly from machines into the cloud, you’ll save scientists time and reduce errors. Ensure all the important context is captured by leaning on software like Ganymede to help associate metadata with a tagging structure (more on that below).
How Ganymede Does It: We automatically organize files during capture of the information. Our Universal Connectors allow us to put agents on machines that “listen” to instruments 100 percent of the time, automatically grab information, label and organize it, and move it into cloud storage. We then feed it back into ELNs, clean, properly labeled, and ready to use for analysis. We even capture all the metadata from every source, so scientists have all the context they need for every piece of data. Learn more about Agents here.
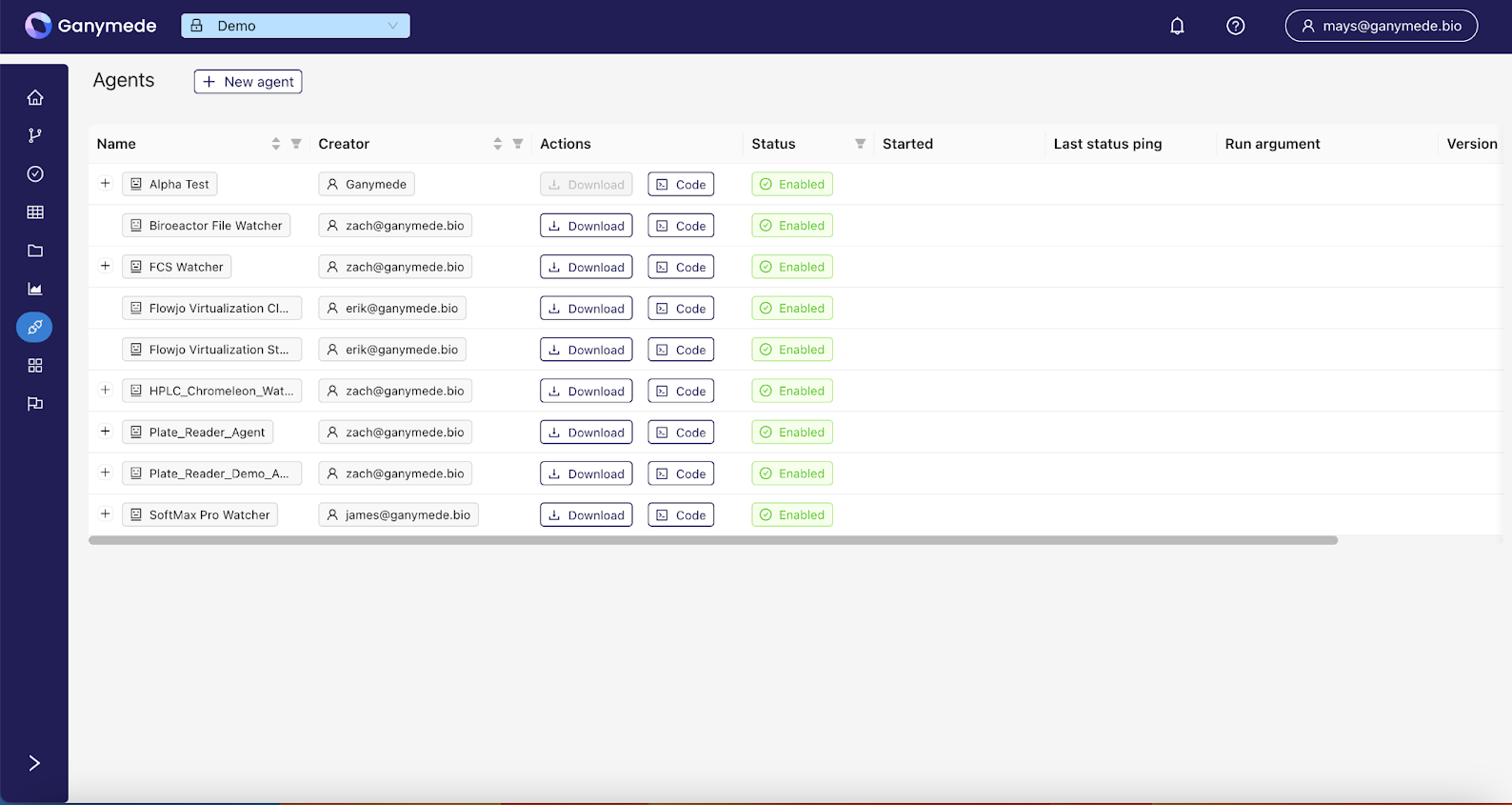
Focus on flexibility
Because we’re all so used to tools like Sharepoint or Windows’ file browser and the traditional folder structure for organizing files, that’s the approach many labs take to storing scientific data. Scientists often drag and drop instrument data into a folder structure, such as: year, month, date, machine, etc.
But what happens if you want to find information about experiments run on an individual machine—say a specific mass spectrometer—across a range of time? You have to literally click into every folder to manually gather information, which will take up hours of time and may not even turn up all the necessary files. Worse, those folders don’t have any metadata associated with them.
Data must be stored with a flexible hierarchy. Give people a way to discover information, rather than needing to know ahead of time exactly what they’re looking for. With a strict folder system, this just isn’t possible.
We encourage companies to move on from legacy filesystems and to embrace tagging. By storing data in a cloud-native SDMS, labs are able to tag information dynamically with valuable metadata, such as:
- The source of the data, such as specific instruments
- Lot numbers from external vendors like cell banks
- Years, dates, and times
- Locations, such as different labs, buildings, etc.
- The scientist(s) who conducted the experiment
- IDs from external lab software systems
- Etc.
Because tags can be customized for anything, they allow labs to be highly specific and flexible about what metadata they track and what they make searchable. Freed of folders, labs can easily see all their data, find what they need, and respond quickly to questions or problems. Traceability should not be an afterthought. For example, finding out which experiments may have been affected by a bad cell lot should be as easy as clicking a tag and making a list.
How Ganymede Does It: We have tagging built into our platform. Companies can choose from a set of standard classes of tags, such as instrument ID, or create their own custom tags. We also import tag types from other software, like Benchling.
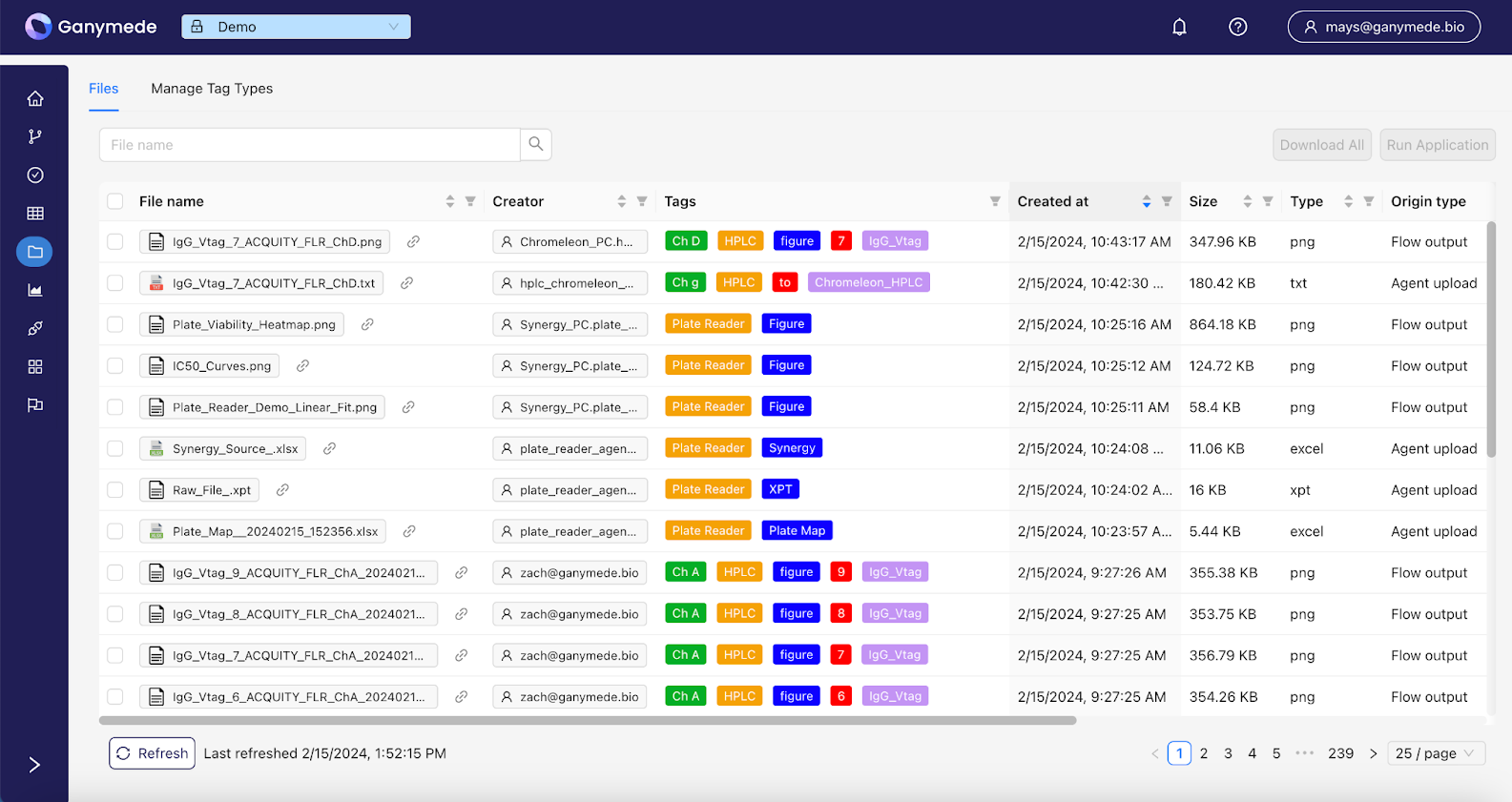
Scientific Data Management is a Key Part of Digitalization
For any modern biotech company today, ELNs are the baseline. No one can operate a lab without them. But an ELN with unstructured data isn’t enough. Organizations also need a robust scientific data management system to filter data, structure metadata, and save everything in the right place.
Companies will struggle to grow without a great data strategy to give form to data collection. While taking control of scientific data management may be intimidating, it’s a crucial part of digitalizing the lab, maturing data practices, and building a lab of the future.