Digitalization Accelerates, Data Takes Center Stage in Shift to AI, and Talent Upskills: Our 2024 Biotech Data Predictions
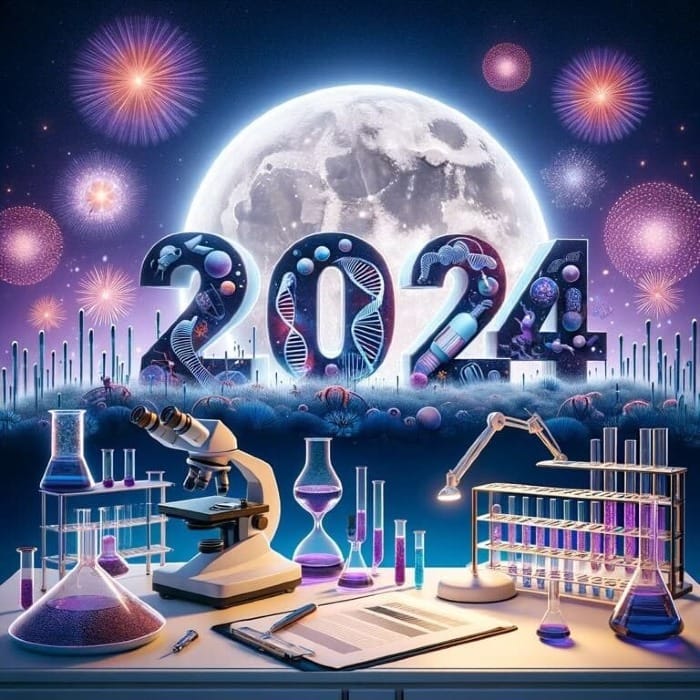
The New Year is here, and many folks in biotech are probably ready to say goodbye to 2023. Coming out of the pandemic, the last few years haven’t been easy on the life science industry.
We’ve seen layoffs, waning funding from VCs, and a drop in biotech IPOs. As we kick off 2024 and life science leaders talk business at the JP Morgan Healthcare Conference, many of them are keeping a keen eye on the future. They’re asking: What’s going to happen? What will it mean for our company? What do I need to be thinking about in the next twelve months?
Despite the celestial origins of Ganymede’s name, we’re far from fortune-tellers or horoscope writers—we can’t predict the future. But we do have a few thoughts on where the industry might go in 2024, especially when it comes to lab and manufacturing digitalization, cloud data tooling, AI, and instrument data. Here are a few trends we’ll be watching this year.
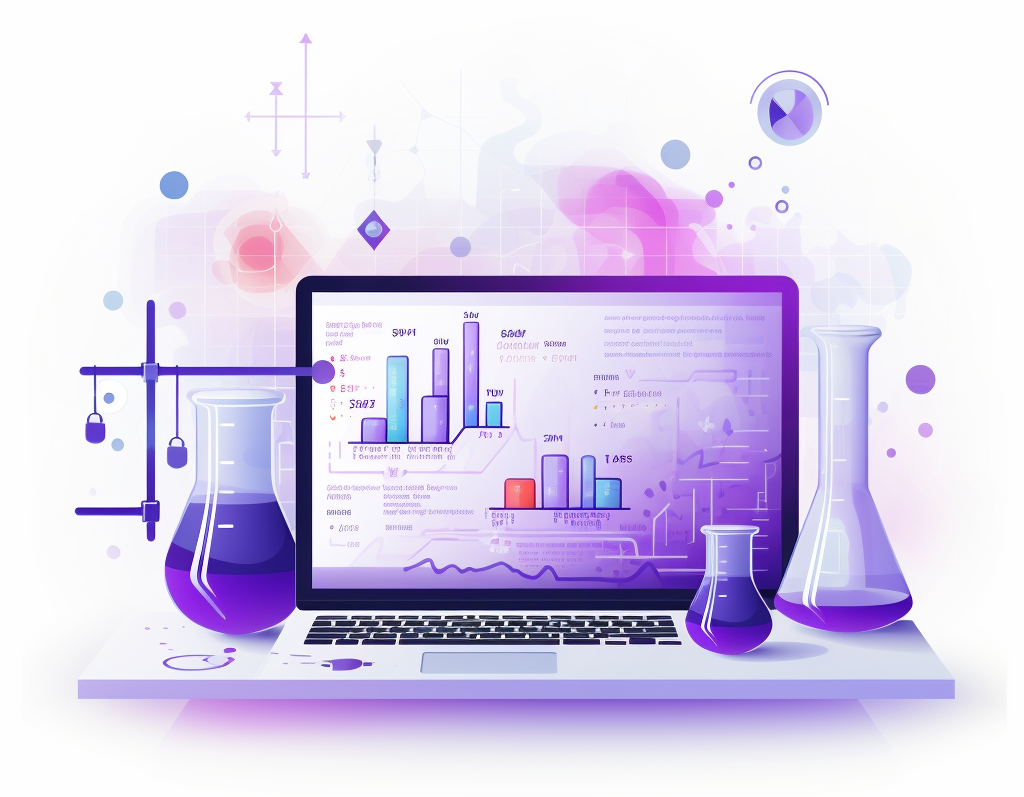
Lab Digitalization Will Continue to Gain Momentum
In 2023, several data-related scandals roiled biotech headlines. Even beyond these surprising stories, biotech has long grappled with reproducibility issues, where lab data can’t be independently replicated by others.
Both data scandals and reproducibility questions stem from a common problem: poorly managed and organized data. In many cases, companies and scientists can avoid problems by getting their data in better shape by adopting FAIR principles (Findable, Accessible, Interoperable, and Reusable) across the entire organization. When data is well-tracked, stored, and organized, it’s much easier to ensure the integrity and quality of information, collaborate, and adopt new technologies.
However, many biotech companies are still early on their data journeys. When it comes to modern data management, we often note how far behind the life sciences are compared to others. (In fact, Nathan recently wrote about his data-related observations from a stint in the finance industry on our blog). Many labs operate with siloed data, sometimes even tracking information by hand on pen and paper.
Over the last few years, this has started to shift. Companies are embracing cloud computing and cloud-native solutions for software including:
- electronic lab notebooks (ELNs)
- laboratory information management systems (LIMS)
- manufacturing execution systems (MES),
- and other tools.
Scientists increasingly need to code as part of their daily work, and biotech companies are hiring a different kind of scientist in parallel: data scientists. As the Wall Street Journal reported, pharma giant J&J hired 6,000 data scientists. Companies are moving from paper, to paper on glass to, eventually, automation. In short: biotech companies are digitizing their labs.
2024 will be an important year for lab digitalization, even beyond big pharma companies with deep pockets. Despite tough market forces, at Ganymede we speak with many mid-sized biotech companies pushing toward the clinic. Some may even be eyeing a (hopeful) resurgence in M&A activity and looking to formalize documentation for due diligence requests. Regardless of their reasons, these younger companies are looking at bringing their labs in line with good manufacturing practices (GMP).
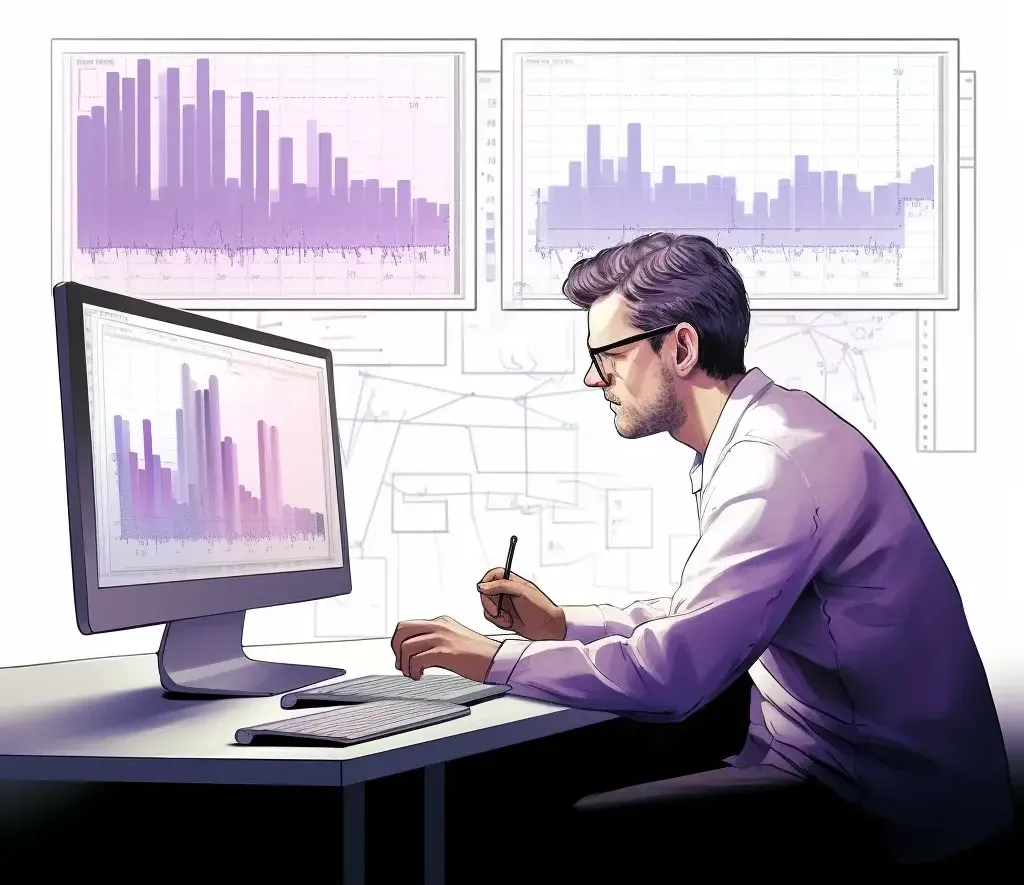
Companies Will Realize AI is All About the Data
If you’ve clicked on a biotech newsletter anytime in the last few years, you’re sure to have seen one word over and over again: AI.
Jumping on the artificial intelligence (AI) bandwagon, biotechs poured resources into bringing the power of algorithms to drug discovery and development. In fact, the WSJ article we linked above speaks about J&J’s focus on and optimism for AI in drug R&D.
Unfortunately, we’ve also seen many of these AI-fueled companies stumble in the last year or so. As Endpoints News noted in October 2023, many of the first “AI designed” drugs have fizzled out.
At Ganymede, we weren’t totally surprised to see this happen.
AI models are only as good as the data they have and the parameters they work within. AI doesn’t function with freeform concepts or without any preconceived notions. Successful examples of AI require well-defined datasets and a pre-existing concept of what the algorithm should be looking at. This is difficult to do in biotech, because each physical experiment provides its audience only a small window of visibility into the complexity necessary for developing a successful therapeutic.
In 2024, life science executives will shift their attention from onboarding new AI tools to ensuring strong data foundations to facilitate higher-impact AI in the long-term. Rather than trying to impose AI approaches—such as large language models (LLMs)—onto biotech, companies will go back to first principles for both science and data. They’ll re-examine how they conduct experiments, the organizational workflows needed to accomplish their goals, and what sorts of data infrastructure/management tools enable those activities.
Once the right workflows, automation tools, infrastructure, and data management practices are in place, AI can be incorporated into models in strategic, domain-specific ways where it will truly add value.
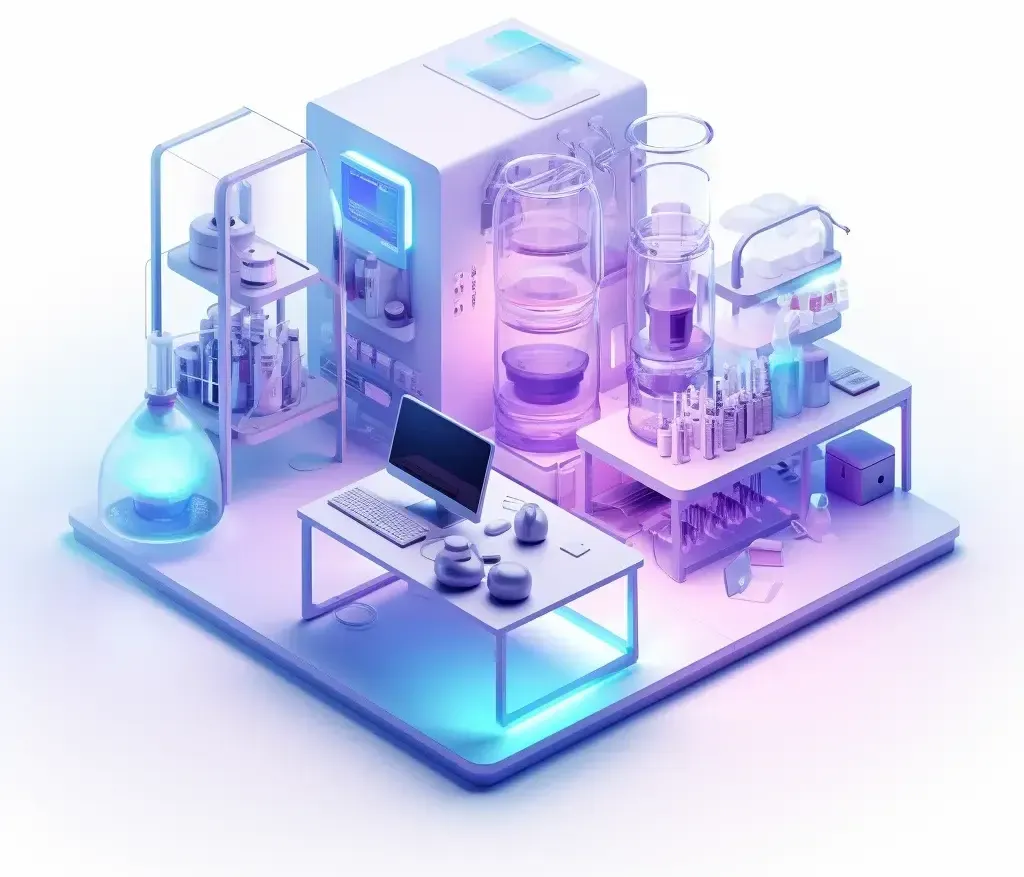
Science + Project Management Skills > Scientific Know-How Alone
For years, postdocs have faced tough prospects in academia; there simply aren’t enough tenured jobs to go around. As a result, more and more Ph.D. students have turned away from university labs and found employment in industry. According to STAT News’ coverage of a recent National Science Foundation report, only a little more than half of PhDs—53 percent, to be exact—planned to do a postdoc.
However, when these Ph.D. wielding scientists take jobs in biotech companies, they’re not really prepared for it. Neither are the scientists who, even if they’ve completed a postdoc, still make the jump to industry. Both groups have classic academic science skills, but biotech companies aren’t academia—they’re for-profit businesses, and they prize different abilities and talents.
Take, for example, how science actually happens. In universities, making discoveries is often a lone wolf endeavor. However, in industry, biotech R&D is a team sport. Scientists work in complex lab environments with dozens of machines and colleagues under significant resource pressure. After all, biotech companies are on the clock to deliver a product that’s expensive to create, has little room for error, and must shift from the blank space of early discovery toward a formalized development and manufacturing process. In contrast, the academic mindset is exploratory and open-ended.
Scientists who can master large-scale project management skills will have a real opportunity in 2024. As budgets tighten and the life science industry stabilizes after COVID-19, companies will want, more than ever, scientists who can work well in teams and deliver results on a timeline. And as digitalization continues to trend in biotech, companies will hold data know-how in higher regard than ever, too. Companies will value both scientists and managers who understand:
- How to capture data with a systems mindset, with consistent context (e.g. related literature, experimental results, methods, conditions, instrument settings)
- How long it takes to process data and how that data is processed
- The different tradeoffs, now and in the future, made with different choices of how to store and process data
- The institutional value of capturing and scaling data beyond any one individual’s work
This mindset requires not just an understanding of code and data management, but also “softer” skills like effective collaboration, workflow management, and more.
For postdocs or Ph.D. candidates looking to move into industry, it will be more important than ever to think outside the beaker and invest in project management and technical collaboration skills. By combining the ability to not only write analytical code but write code that others within their company can use, reuse, and understand, scientists gain a significant advantage.
This isn’t to say that Ph.D candidates or postdocs need to become software engineers. Far from it. However, they should adopt engineering principles of reusing code across the organization. This sort of project management mindset will also benefit AI-centric and collaborative efforts within biotech organizations; well organized information leads to better datasets both for AI models and cross-team collaboration.
2024: A Year of Opportunity
Several years out, the smoke is finally clearing from the pandemic. While there are many unknowns in the biotech market, 2024 is a year of opportunity for biotech to get their data in shape, adopt FAIR principles, modernize their infrastructure, and become more digitally mature. Most biotech companies already use some form of modern, cloud-native software and infrastructure in their tech stack. We’re excited to see this digitalization journey continue and even accelerate in 2024 and beyond. There are amazing opportunities awaiting discovery in lab data—we just need to get the right tools in place to find them.
Take Control of Your Lab Data in 2024