Is Biotech Behind the Times? Four Data Lessons that Biotech Can Learn from Fintech
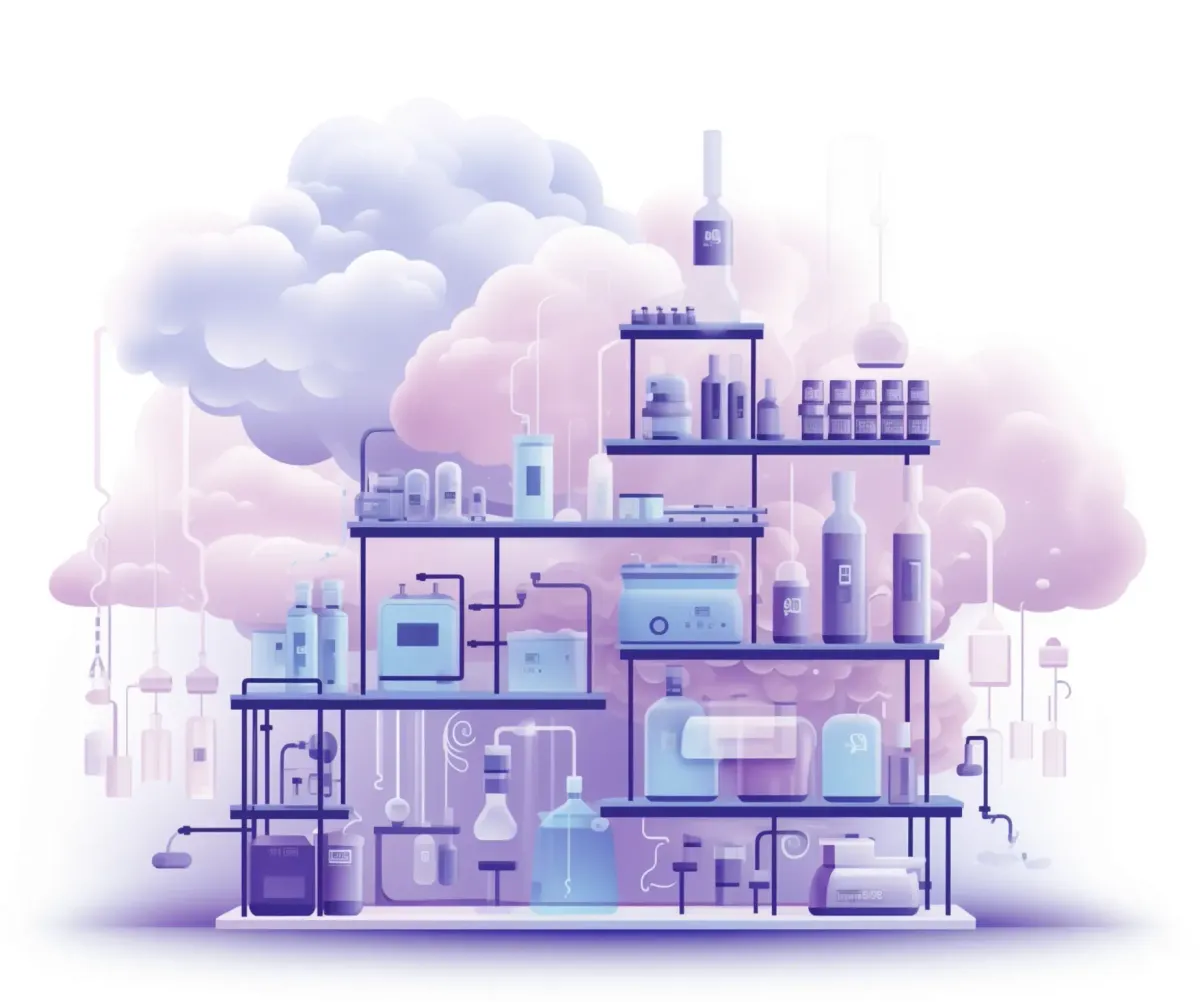
On the surface, the life science and finance industries don’t appear to have much in common. Biotech conjures up visions of beakers and petri dishes, while finance evokes an image of endless spreadsheets, stock tickers, and office cubicles.
However, there are actually more similarities between these industries than people imagine. My own career has straddled these two worlds; I started in the financial industry before moving into biotech, and ultimately founded a company to disrupt scientific and manufacturing data. Comparing my experiences in both industries, I’ve found striking commonalities. Both are industries with highly-sensitive data and IP. Both face complex regulations. Both are, generally speaking, process-oriented. And both are prime examples of industries where software can have—or in the case of finance, has already had—a huge impact.
The Biotech Data Gap
One of the biggest differences between the finance and life science industries is that biotech and pharma are 10 to 20 years behind finance when it comes to data and weaving software into their business. Since the 1970s and 1980s, the finance industry has embraced software wholeheartedly, giving rise to its own category of fintech SaaS, PaaS, IaaS and other companies serving the field. Data is ubiquitously available in finance companies, and it’s incredibly liquid. For example, almost all vendors provide data via web APIs, and almost every piece of data is stored in a clean or open format.
Biotech, on the other hand, has not made such a large, data-forward leap. That might seem surprising, but despite having incredibly advanced science, biotech is a laggard on enterprise software, data strategy, and infrastructure. While there is plenty of modern software in the operational functions of biotechs—like finance and HR—most people would be shocked by how old school things are in the labs, where the actual science happens. Even in 2023, many scientists still record data from their experiments by hand on pen and paper. They do analyses in Excel, or haul data around on USB keys. In other words, in some pockets of biotech, it still feels like the 1990s or early 2000s. The “Sneakernet” still dominates over the internet, and little is online.
This gap between the fields of finance and biotech is largely driven by the complexity of life science data. Financial work often revolves around a core business that has high throughput with a known data structure, unitized by dollars. In contrast, biotech is fueled by people doing R&D (and therefore trying new things) on human biology, which is a monumental tangle of evolution. This means that data is structured differently between every single biotech company and changes even month-to-month—and it needs to be this way, because that’s how the scientific process works. It’s ever-evolving and changing. No two experiments are exactly the same.
As a result, little biotech data can be formally structured beyond Excel spreadsheets or one-off, fit-for-purpose analysis apps with high degrees of human judgment involved. There’s also little in the way of data science or automation that can be achieved in most biotech wet labs. By extension, there are weak incentives for data sources—primarily lab instrument makers—to provide clean APIs or any good way to avoid manually transcribing data.
However, this reality is shifting. Biotech companies are recognizing that the industry’s delay in adopting advanced data infrastructure, strategy, and infrastructure is standing in the way of science itself. They’ve begun to recognize how cutting-edge data management can uncover R&D discoveries faster, speed time to market, and help patients in need sooner. In fact, that’s a huge part of why I moved from fintech into biotech and eventually started my own company, Ganymede—to make data-related improvements that help biotechs do better, faster science.
So while finance and biotech may seem worlds apart, there are valuable lessons that fintech can teach biotech when it comes to data and software.
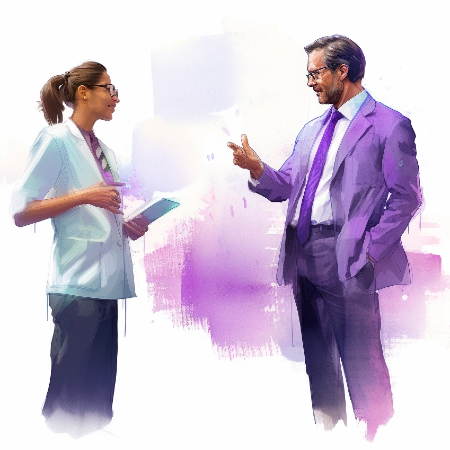
Lesson One: Minimize Bespoke Infrastructure In the 1960s and 1970s, finance was one of the first industries to invest in mainframe infrastructure. They built and maintained their own custom-built computers and servers. This was hugely expensive and inefficient. Fast forward to the 1980s, those same computers and servers could be bought off the shelf in the PC revolution. By the early 2000s, PCs were ubiquitous in every home—saving companies money and paving the way for cloud storage, artificial intelligence (AI), and other advanced tech.
Biotech is, in some ways, in the pre-1980s phase when it comes to scientific data. Wet lab data is incredibly messy, relies massively on manual work, and resists standardization. Every lab experiment is different, and there’s not a lot of reusable infrastructure from one lab to another. Right now, biotechs spend a huge amount of time and money on expensive, complex, and bespoke company-wide digital transformations and data lakes, which are the equivalent of centralized mainframes in the data world.
This is not to say that wrangling biotech data is an intractable problem. Biotech is so complex that labs will always require some level of bespoke work, whether that’s infrastructure, coding, or tools. However, labs can standardize how they store and access their data. As they embrace standardization, more off-the-shelf offerings will emerge.
In fact, the data-generating systems that scientists use in the wet lab are now starting to report data via things like web APIs rather than files, opening up the beginning of the “PC revolution” local to the lab. We’re already seeing solutions leapfrogging forward, such as Benchling for electronic lab notebooks, Veeva for clinical data and drug commercialization, and more.
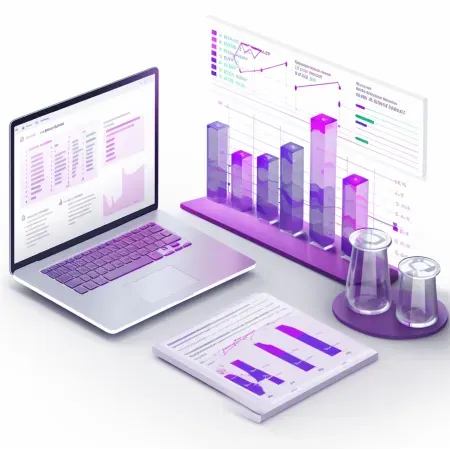
Lesson Two: Automate, Automate, Automate (and then Automate More)
Most finance companies have also invested heavily in the last few decades in automation. They’ve standardized certain sets of human activity, built out decision trees, written scripts, and then tracked every speck of data possible. Today, you’d be hard pressed to find a fintech lender without an AI underwriting capability, for example. It’s become table stakes, replacing what 40+ years ago would have required huge teams of humans managing paper by hand.
Biotech can also improve their data quality and leverage automation, albeit in different ways. While some level of biotech data and workflows will always be too complex for standardization and automation, there are still opportunities for improvement. For example, scientists often spend a lot of time on repetitive, non-scientific tasks, such as exporting data from an instrument into an Excel spreadsheet. Biotechs can take a page from finance and automate this sort of busywork.
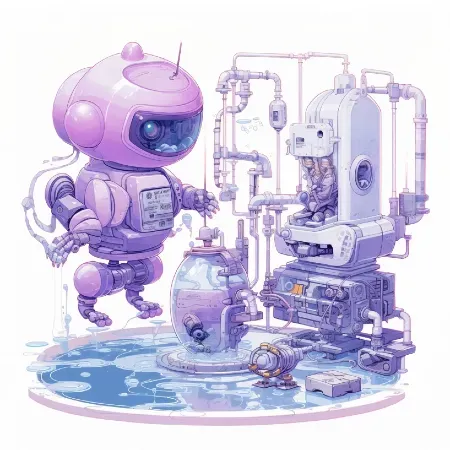
Lesson Three: Garbage in, Garbage Out
Of course, all this standardization and automation requires the same thing: high-quality, clean data. Automation works best with clearly-delineated, standardized, and large sets of data. This is something the finance industry has excelled at, making data clean and generally available. This wealth of data that’s easy to analyze and easy to use has fueled all the automation discussed earlier.
Biotech has messy data, often as a result of the practices I mentioned above, such as transporting data from scientific instruments to PCs via USB keys. Even when instruments do come with APIs—which is rare—the data is often locked in specialty formats, labeled inconsistently, or is missing metadata. Biotechs can automate much of this manual work away, as mentioned earlier. However, that data needs to be automatically ingested into a data platform in a way that is consistent and makes information easy to find, access, combine with other information, and analyze. Good data leads to good insights, good analysis and good discoveries.
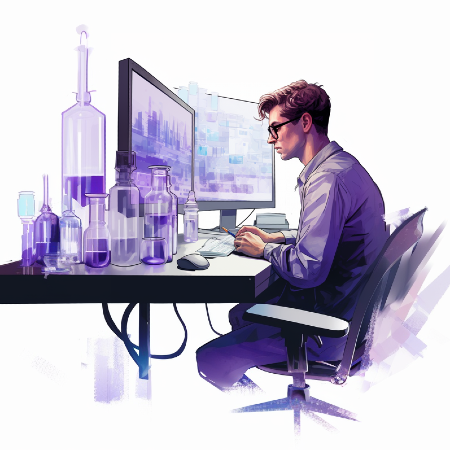
Lesson Four: Turn Everyone Into a Data Scientist
Once companies have invested in data infrastructure (point one), automation (point two), and clean data (point three) they’ll have a lot to work with. To get the most out of that data, companies should equip everyone in the company with the tools to access, analyze, and benefit from that data. This is common in finance. Analysts and other similar employees have business intelligence tools at their fingertips to take a look at data and uncover insights. For example, a credit card company employee could quickly correlate a user’s credit score data with the geographical location of purchases using simple low-code tools and SQL.
While scientists are not empowered to code today, biotech companies can take a path similar to fintech to change this. With the right tools and training, scientists can query across different types of files or datasets and make the most of their data. By turning everyone into not just a scientist, but also a data scientist, biotech organizations also start to make headway with AI and ML—another area where other industries have lapped the biotech space.
Biotech companies work with some of the most valuable data in the world. The experiments they conduct lead to new treatments and cures for patients. These ground-breaking companies need tech as advanced as their life-changing and life-saving science. If data from labs were better organized, think of how much faster those groundbreaking discoveries could be made—and how much value these companies could create. While the biotech industry may be at the beginning of its data journey, a few well-placed lessons from more established industries, like finance, could speed things up. The next discovery is just a few bits and bytes away.