2023 under the microscope: people management is a key data challenge to solve
The new year is just around the corner. We sat down with industry scientists to walk through how we can improve scientific data management in 2024.
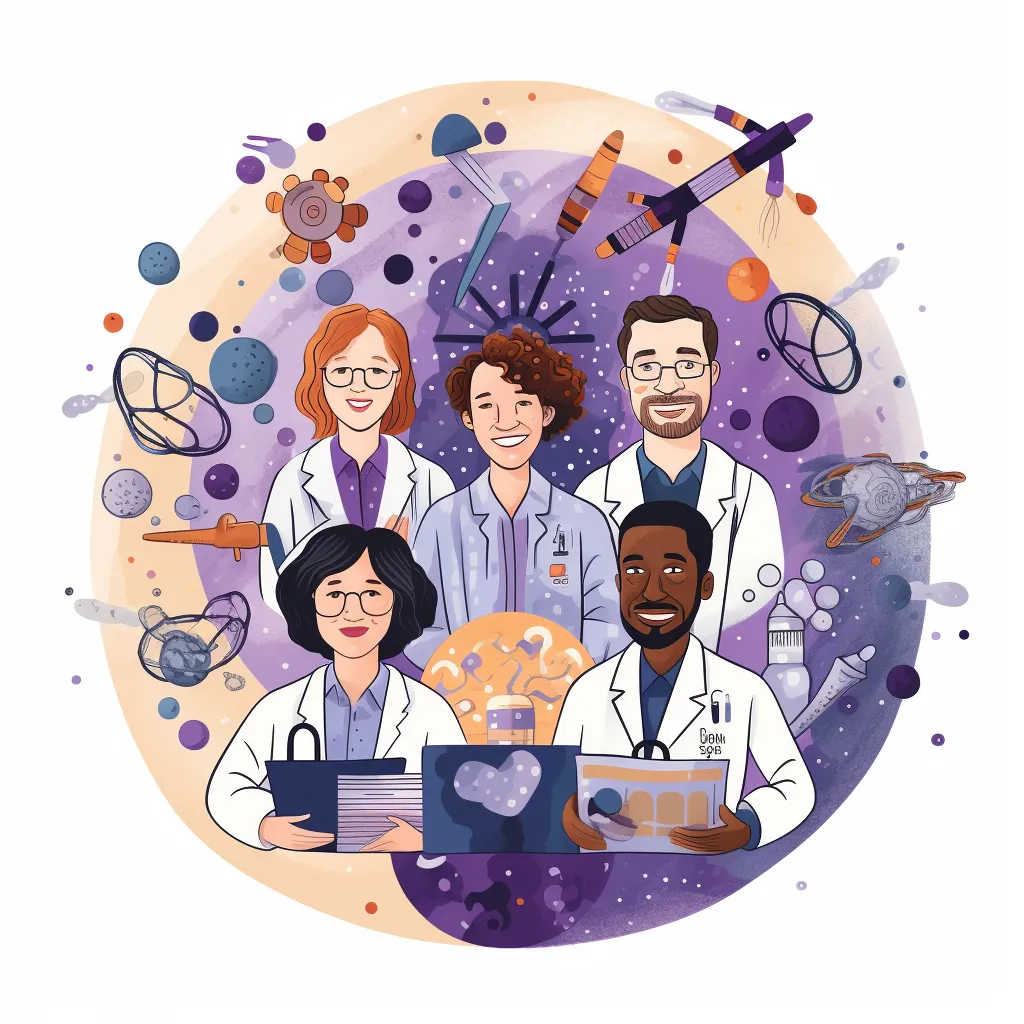
Earlier this year, Ganymede Cofounder and CEO Nathan Clark sat down with two lab automation leaders at the 2023 Future Labs Live (FLL) US convention:
- Victoria Mander, Scientist and Automation Team Lead at Janssen
- Karthik Raj Konnaiyan, Senior Laboratory Automation Engineer at Tolmar
They geeked out about all things automation, data, and digitalization in the life sciences, touching on a range of experiences and trends in the space. As 2023 draws to a close and companies are thinking about what to focus on in 2024, we wanted to expand on some of these ideas. In this post, we’re sharing four key, data-challenges companies should prioritize resolving in the year ahead.
Most surprisingly? The challenges are actually related to people management, and not data management—read on for more!
1. Invest in data talent development
Most of the people touching code in labs fall into two categories:
- Bioinformaticians who are highly skilled at building bioinformatics pipelines, but not accustomed to handling data from all of the other instrumentation in a lab
- Developers, programmers, and software engineers who’ve moved into the life sciences from more of a traditional tech/data science background
Though they come from different backgrounds, both of these groups of people share something in common: they haven’t really been trained for whole-lab scientific data management and automation. Yet bioinformaticians will often find themselves responsible for digitally connecting all of their organization's lab data, especially if they’ve joined a small and scrappy biotech. On the flip side, developers moving from tech to biotech are seasoned experts when it comes to code. But science? Now that’s new. They need more training on the nuances around life science data, with all of its complexities and quirks.
And, you can't really look to the life scientists for solutions - most haven’t received any formal instruction in coding. Of course, even if they can't code, scientist involvement is critical to any successful digitalization and automation effort.
As the demand for automation and artificial intelligence (AI) grows in the life sciences, both scientists and IT engineers will need to bridge the gap between tech and biotech skills. Both of these areas are quickly evolving, and the nature of scientific research adds additional complexity— no two companies, experiments, or drug programs are alike.
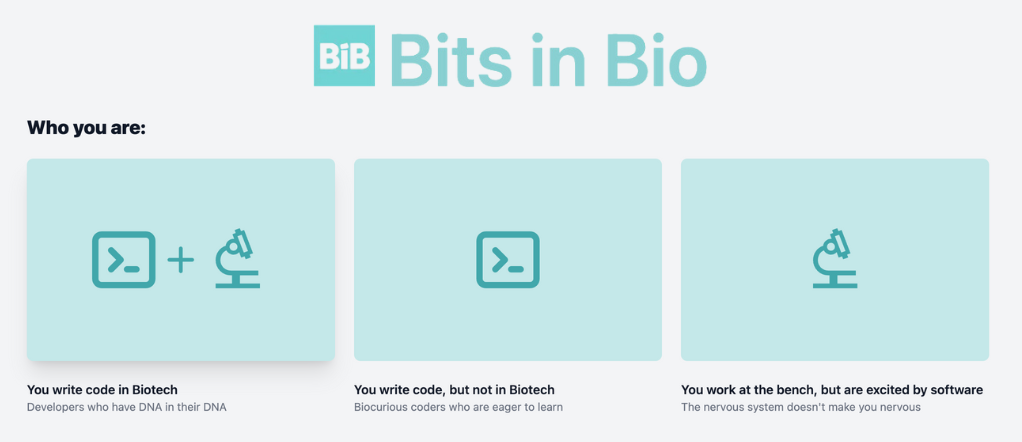
As Victoria from Janssen put it in her conversation with Nathan at FLL: “[Data Automation] is a rapidly developing field and the needs are constantly changing based on the customer, so how do we train our people so we’re not reinventing the wheel every single time that we try to automate something?”
Unfortunately, there’s no formal training available (yet!) around data management and data science for biotech. That means organizations are responsible for providing that training to their staff. In 2024, companies should provide more access to knowledge, training, information, and room for collaboration to both their scientists and developers.
Some great places to start are:
- Bits in Bio - an immersive, free, and rapidly growing community of anyone interested in the intersection of science and technology. Make sure to join their highly active Slack community.
- Coursera - for both scientists and developers looking for a range of classes/courses across coding and biotech.
- Biotech Primer - great for non-scientific folks looking to beef up their life science knowledge on a range of topics related to the full life cycle of drug development, such "Preclinical Drug Development 101" and "Biobasics 101: The Biology of Biotech For The Non-Scientist." These “primers” come with a cost though, much like the ones you use in the lab.
- codeacademy - for scientists just starting on their coding journey and want to learn the basics with easy to follow along with modules. We suggest taking up the Data Science skill path, which opens up the power of SQL to scientists.
- Gihub - we’re big fans of open source tools, and Github has >100 public repositories you can explore and use in your scientific analysis.
2. Build teams with diverse skill sets and backgrounds
Across industries, everyone knows the key to success is building the right team. Unfortunately, when it comes to data in the life sciences, rarely is there an administrative approach towards building comprehensive scientific data teams. In pharma and biotech, the people who touch data—scientists, developers, engineers, and others—are scattered across different units within a single company. This hodgepodge approach leads to information and data silos, lack of standardization, and inefficiency.
In 2024, life science companies should look to thoughtfully build dedicated teams to handle data. They should combine folks with a broad range of skill sets. We have a model to follow already: This is how bioinformatics and "-omics" labs and companies already handle data. To fully understand their company’s data, most dry lab bioinformaticians work hand in hand with wet lab scientists—they essentially form a symbiotic relationship.
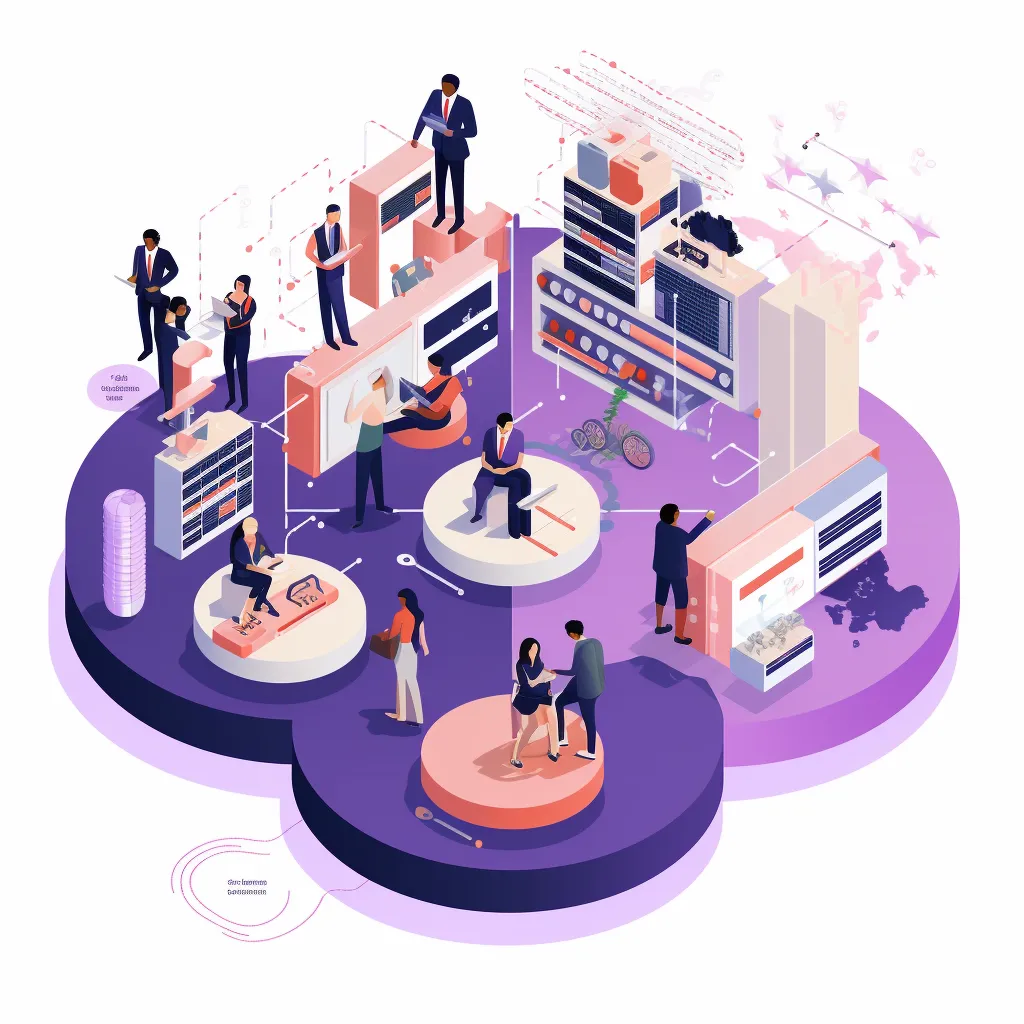
What could this look like for the broader industry? For companies dealing with a relatively standardized workflows, a team could include:
- Traditional scientists
- Automation engineers
- Software engineers/IT, and
- Data scientists
And of course, no matter the team, life science companies should be thoughtful about diversity within their organizations. At FLL, Victoria noted that she’d like to see more representation for women within automation, robotics, and data science. In her words: “Diversity brings diverse perspectives. And the more diverse perspectives, the better outcomes you’re going to have ultimately.”
3. Use automation to shift everyone's time to higher-value work
Lab automation has demonstrated tremendous impact on the life sciences. Data automation, on the other hand, is still a fairly new concept to most labs. Scientists, for example, still spend an inordinate amount of time on manual data processing tasks like data entry.
In fact, we’ve heard from Ganymede’s customers about “ELN days” and even “ELN months.” Yes, you read that correctly, an entire month, usually at the end of the year, dedicated to fully updating an ELN. Scientists save up all their data and then spend countless hours manually porting data on USB keys or shared drives from instruments to PCs, copy/pasting data into electronic lab notebooks (ELNs) or Excel sheets, and more.
Automating this tedious, manual work can have huge benefits. Scientists, of course, save time and can focus on more high-value work. However, there’s also huge benefits in terms of data quality (less human error) as well as being able to understand the full context of both data and metadata across the entire lab.
Karthik from Tolmar shared thoughts at FLL about the value of automated data to scientists: “Every system generates its own type of data. You have identifiers like sample type and sample IDs. But still, you want to know on the process side, what configurations you’re using. Because sometimes you do re-testing and sometimes you run the test in a different way to get some certain results, but those things are not recorded properly. But those are valuable information—always you should not only look into the success of the past tests, but you want to learn and understand why it failed and what you tried to do to make it right.”
We sat down with other industry innovators to identify how data and lab automation can be paired together to streamline R&D and give back precious time to scientists.
Let’s make 2023 the last year scientists need to manually port data from point A to point B and sift through raw files to understand experiments. Scientists should spend their time on high-value work—on actual science. Data automation can take excruciatingly tedious tasks off their plates and allow them to focus on contributions that will add more value to the company and be more meaningful for the individual, too. After all, no one gets into science for ELN days… or months.
4. Get your team ready for AI by standardizing and FAIR-ifying your data
While some healthcare industry regulations like HIPAA or security regulations may apply to certain companies, there isn’t necessarily a set of standards for how lab data is structured and stored. This means biotech organizations don’t have a “paint by the numbers” approach to their data.
Without standardization, companies face an uphill battle with their data—especially when they want to bring in new tools and software. This includes AI, which is a buzzy area of interest in the life sciences.
Karthik from Tolmar spoke to Nathan about this at FLL, saying: “You can bring all types of new technology and platforms and everything, but [if] you don’t have a standard where you can consume the data and make sense of it, you are going to spend more time managing and manipulating the data to get it to a model to make sense of it.”
In 2024, companies should think deeply about how to standardize data within their organizations. As a baseline, this data must be FAIR, as defined by GO FAIR.
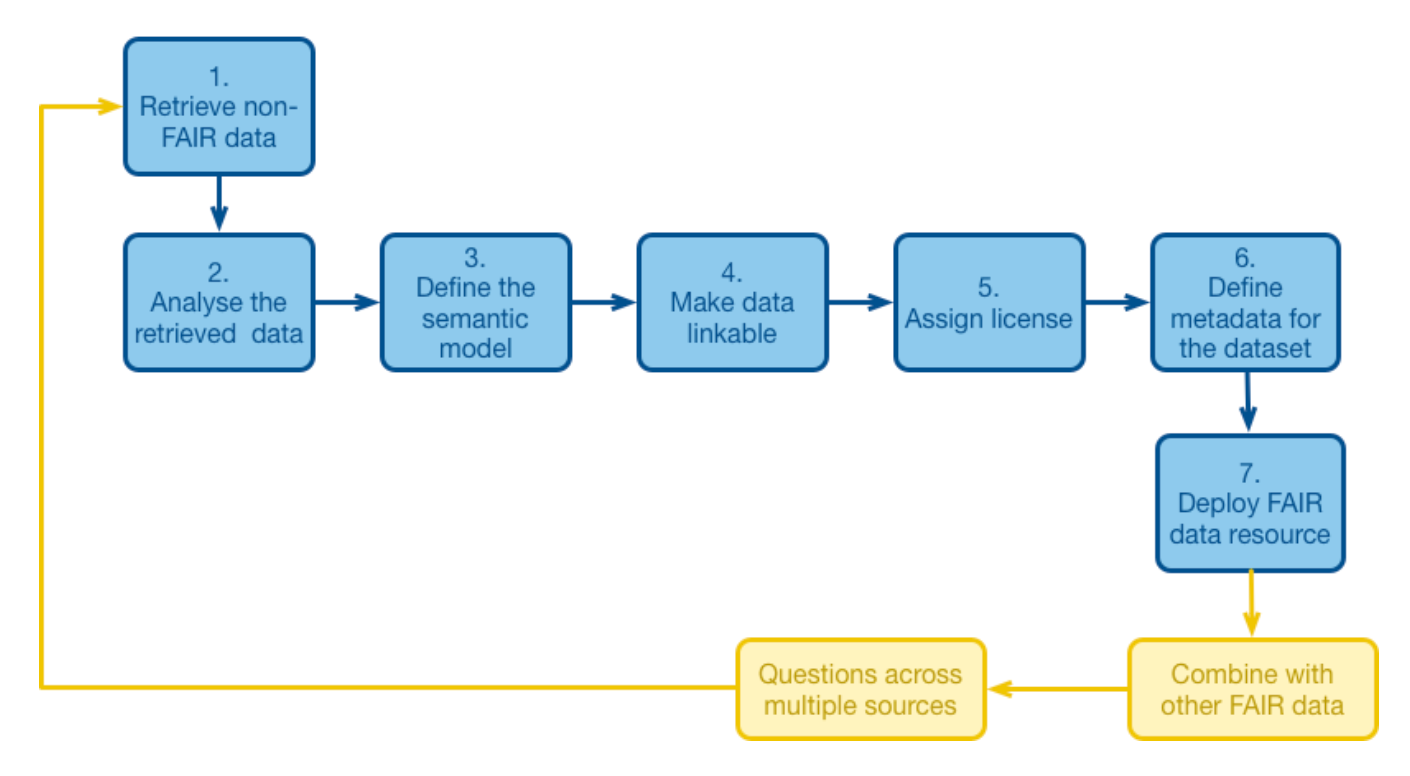
This can be difficult to achieve in biotech, both in terms of the complexity of life science data and the time it takes to properly gather, format, and store information.
At FLL, Victoria from Janssen touched on some of those complexities, saying: “The whole point of science is that we don’t know what’s going to happen, so we have to test it. Oftentimes we get back results we don’t expect. How do we catalog those? How do we visualize that? How do we learn from it? Incorporating that across multiple platforms, multiple instruments is critically important but also time-consuming.”
This points to an important nuance: as biotech organizations standardize the ways they collect, structure, and store data, they should also allow flexibility. Without flexibility for the unknown elements of science, companies risk becoming too rigid and having to reinvent the wheel constantly.
Building a Foundation for Success in 2024
All of the points above have one common theme: they are all about building a strong foundation for success with data. Whether it’s bringing the right people to the table, investing in and growing your team’s skills, or getting data in shape for AI and other new tools, each of the topics above contribute to creating a strong data organization.
And a strong data organization has benefits for everyone: scientists save time, developers and engineers can build more meaningful pipelines, and the whole lab moves faster. FAIR data tends to have a multiplier effect, too. Not only can scientists use it for their analyses, but the whole organization can use that same data to glean insights, too.
So as you think about teams, budgets, and the year ahead, we hope you’ll think about this post—and watch Nathan’s interviews with Victoria and Karthik for more information and inspiration.
Our CoFounder Nathan Clark sat down with Victoria Mander, Scientist and Automation Team Lead at Janssen.
Our CoFounder Nathan Clark sat down with Karthik Raj Konnaiyan, Senior Laboratory Automation Engineer at Tolmar.