The Essential Scientific Software Stack for Data at Every Stage of Drug Development
Understanding the key data problems at every stage of drug development, the software tools that will help you solve them, and how to measure the success of those tools.
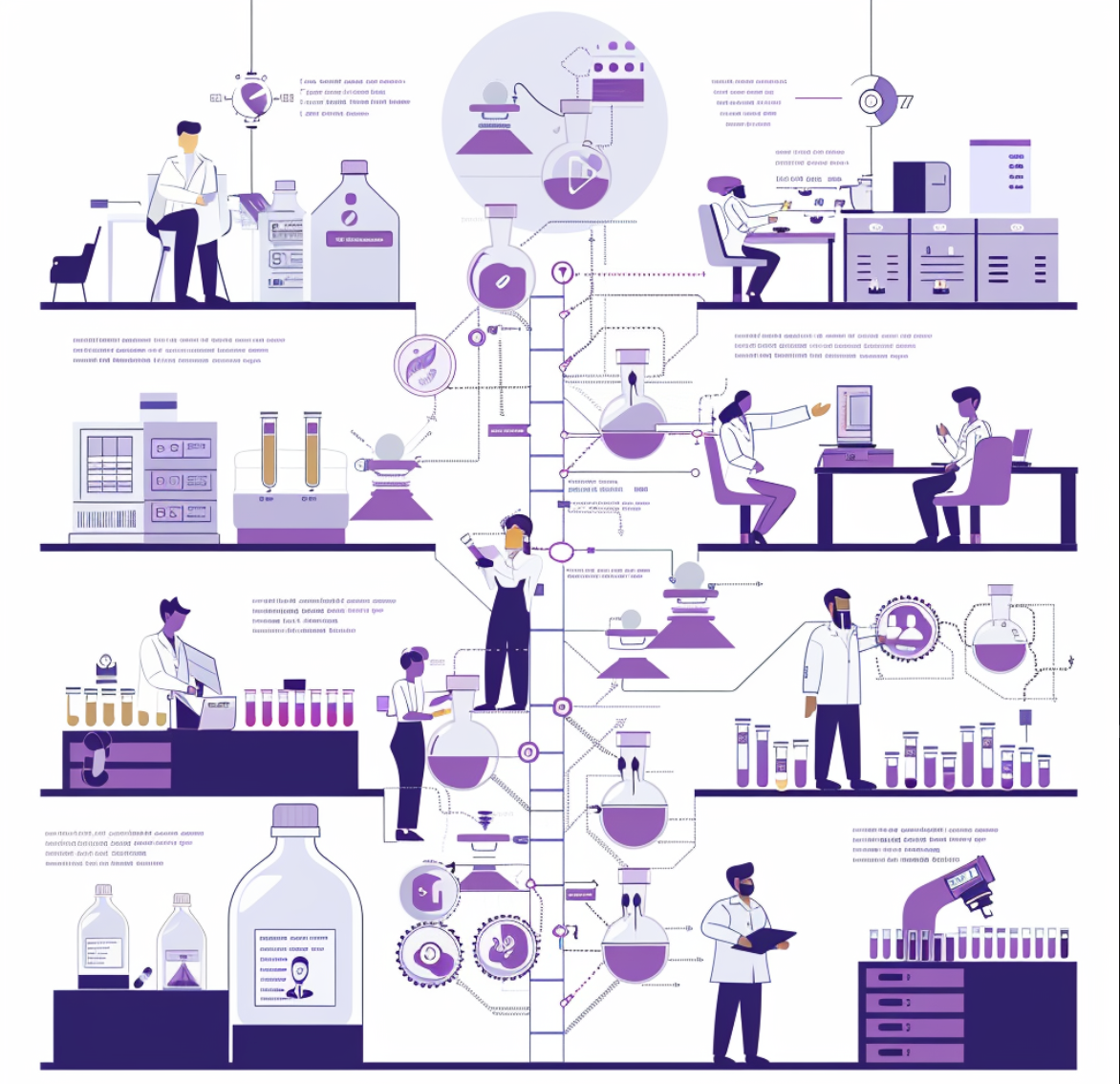
From drug discovery to manufacturing and quality control, every phase of biotech development presents unique challenges and demands specialized tools to overcome them. But it comes down to more than just selecting the right technology for the job. After all, there’s no shortage of promising SaaS tools on the market. Rather, success at every stage also requires ensuring tools are integrated and optimized to deliver value.
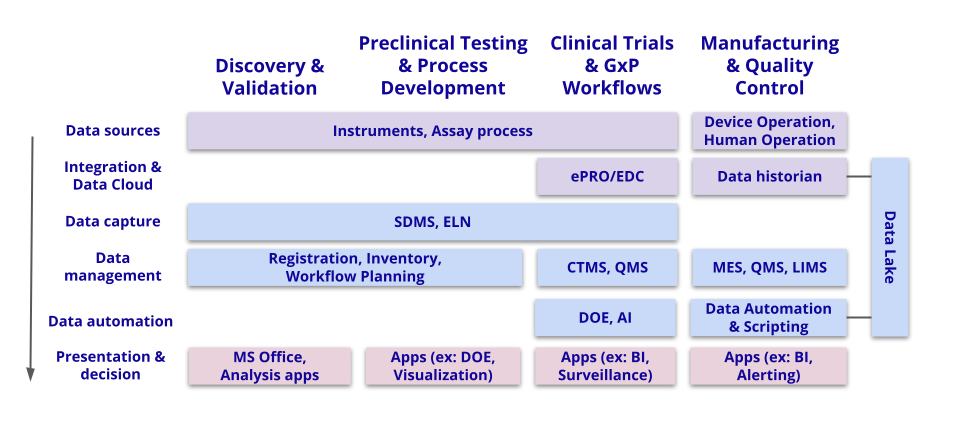
What does that value look like at every step, and how can companies be certain that their investments are delivering it? Let’s dig in.
Discovery & Validation
Goal = Identify a Relevant Target, Find a Functional Lead
At this initial stage, the crucial task is organizing data to enhance visibility, sharing, and analysis throughout experimental cycles. This entails prioritizing the findability (F) and accessibility (A) of data in accordance with the FAIR data standard.
By addressing these challenges, researchers can streamline their workflows, cycle through experiments faster, and foster collaboration—ultimately accelerating the pace of drug discovery.
Commonly-used tools: Biotech companies leverage a suite of software tools ranging from ELNs and SDMS for data capture and management, to inventory and registry systems for resource tracking and management. Meanwhile, workflow planning tools and lab automation orchestrators optimize experimental processes, while screening/analysis tools and omics platforms aid in data analysis critical for identifying and developing novel therapeutics.
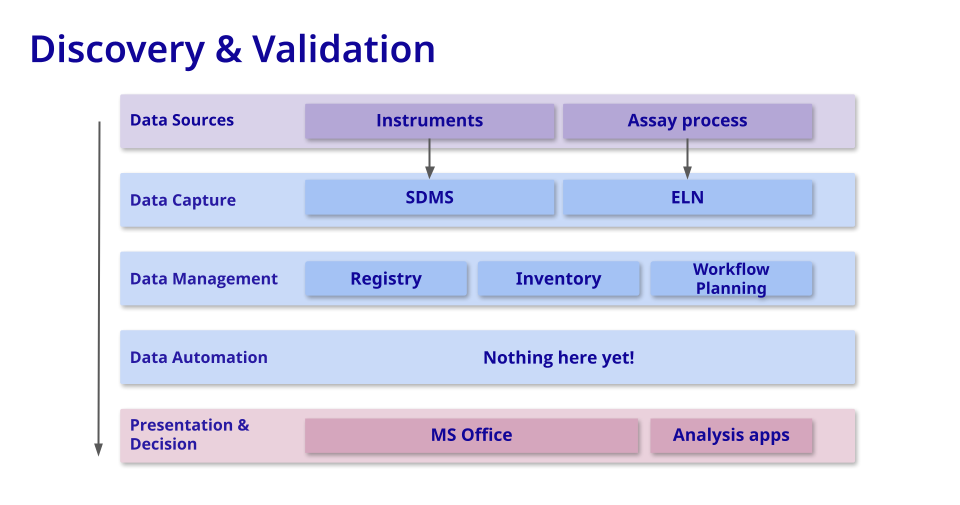
Measuring value: In assessing the value of software tools in the drug development discovery stage, metrics such as tool adoption by headcount, reduction in the reliance on general productivity tools, and improvements in sample throughput and turnaround time serve as pivotal indicators.
By tracking these metrics, teams can effectively gauge the efficiency and impact of software tools, enabling informed decision-making and optimizing the research process for enhanced productivity and outcomes.
Preclinical Testing & Process Development
Goal = A Reliable and Optimized Therapeutic, From Well Plate to Patient
In the preclinical and process development stage of drug development, scaling up processes and managing expanding datasets while preserving data integrity and facilitating centralized insights to expedite development becomes the central challenge.
Here, the emphasis on interoperability (I) and reusability (R) within the FAIR principles becomes crucial, ensuring seamless integration and exchange of data across different platforms and enabling the efficient reuse of valuable information. By prioritizing these FAIR principles, teams can effectively tackle data complexities, optimize workflows, and propel progress in drug development endeavors.
Commonly-used tools: Biotech companies leverage sophisticated software systems like LIMS, integration builders, DOE/PAT systems, data lakes, and IND filing backbones to enhance data management, streamline processes, and ensure compliance during drug development and preclinical research. These systems play a crucial role in optimizing experiments, managing data, supporting regulatory submissions, and facilitating informed decision-making in the pursuit of developing safe and effective therapeutics.
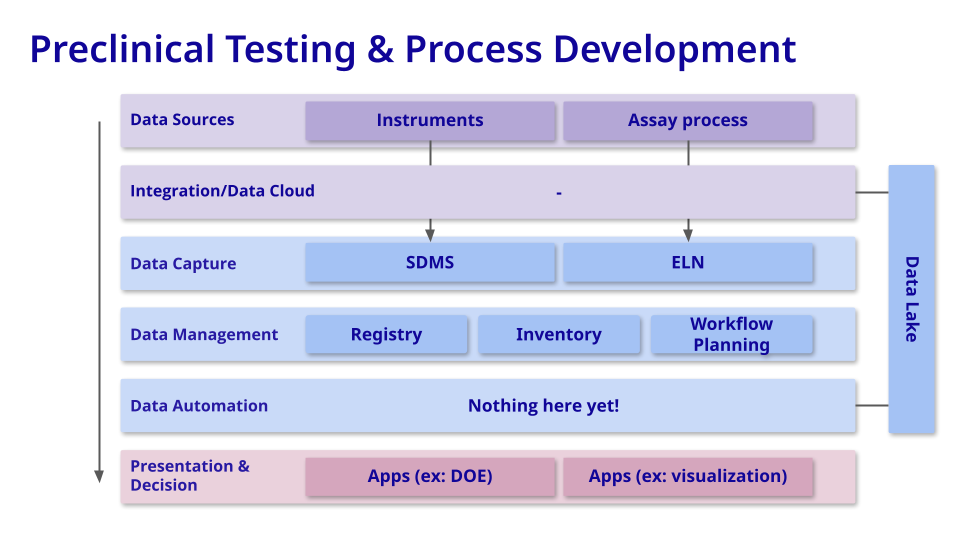
Measuring value: Unlike the discovery phase where you are accelerating through failed candidates and experiments to find promising ones, in this stage success is defined by improving an evolving workflow, validating that your candidates work safely in established pre-clinical models, and starting to scale production up for clinical trials in actual patients. Specifically, we're looking at decreasing the number of experimental cycles required for a stable product, higher batch throughput and consistency, and percentage of all data captured as key indicators of success.
Clinical Trials & GxP Workflows
Goal = Ensuring Patient Safety and Therapeutic Efficacy
In the clinical and GxP stage of drug development, the focus is on ensuring complete process compliance and comprehensive data capture while minimizing reliance on human data entry and discretion. This entails implementing robust systems and technologies to automate data collection and validation processes, reducing the risk of errors and ensuring data integrity throughout the regulatory journey. By prioritizing these measures, organizations can enhance efficiency, accuracy, and regulatory compliance in their drug development endeavors.
Commonly-used tools: During the clinical and GxP workflow phases of drug development, biotech companies rely on clinical-facing tools (CTMS, EDC), trial analytics software, QMS, and V&V backbones to optimize clinical trial management, ensure data quality and integrity, maintain compliance with regulations, and support quality assurance processes. These software tools play a critical role in driving efficiency, transparency, and regulatory compliance in the clinical development of new therapies.
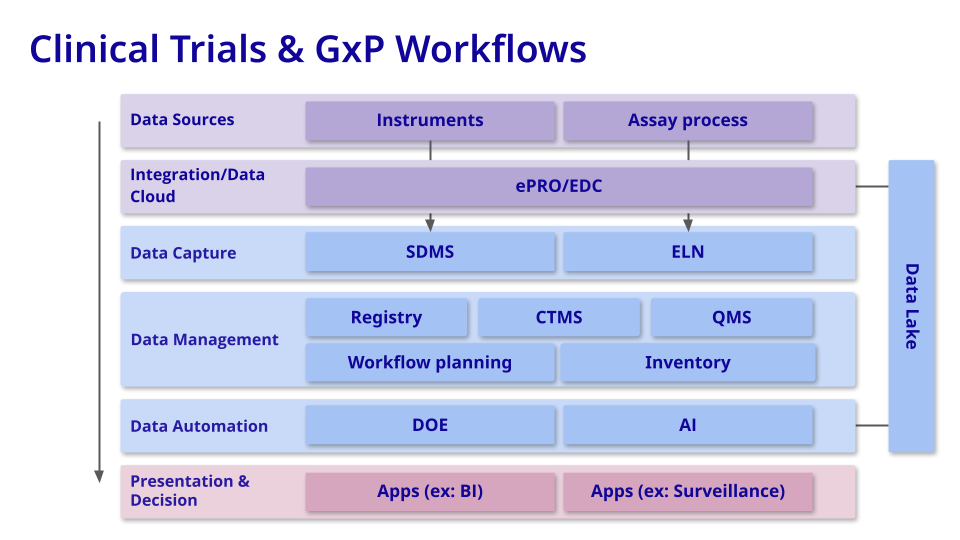
Measuring value: When measuring value at this phase, trial data coverage percentage, validation velocity, percentage of systems and processes validated, and batch production process parameter coverage will be the most critical metrics to review.
Monitoring these areas enables teams to ensure comprehensive data coverage, accelerate validation processes, and validate a higher proportion of critical systems and processes, which increases efficiency and compliance of batch production.
Manufacturing & Quality Control
Goal = Product Integrity at Commercial Scale
Comprehensive data coverage and data integrity remain crucial in the manufacturing and quality control stage of drug development. Implementing automated alerting systems to promptly flag parameters that deviate from acceptable bounds becomes increasingly important as well.
By prioritizing these initiatives, companies can ensure the reliability of their manufacturing processes and uphold stringent quality standards, safeguarding the safety and efficacy of the produced drugs. This proactive approach not only minimizes risks but also enhances operational efficiency in drug production.
Commonly-used tools: At this phase of development, biotech companies use MES for production management, SCADA for process monitoring, visibility & analytics tools for data analysis, and LES for laboratory process automation. These software tools play a critical role in ensuring product quality, process efficiency, regulatory compliance, and data integrity throughout the drug manufacturing and QC processes. They enable biotechs to optimize operations, mitigate risks, and deliver high-quality pharmaceutical products to market.
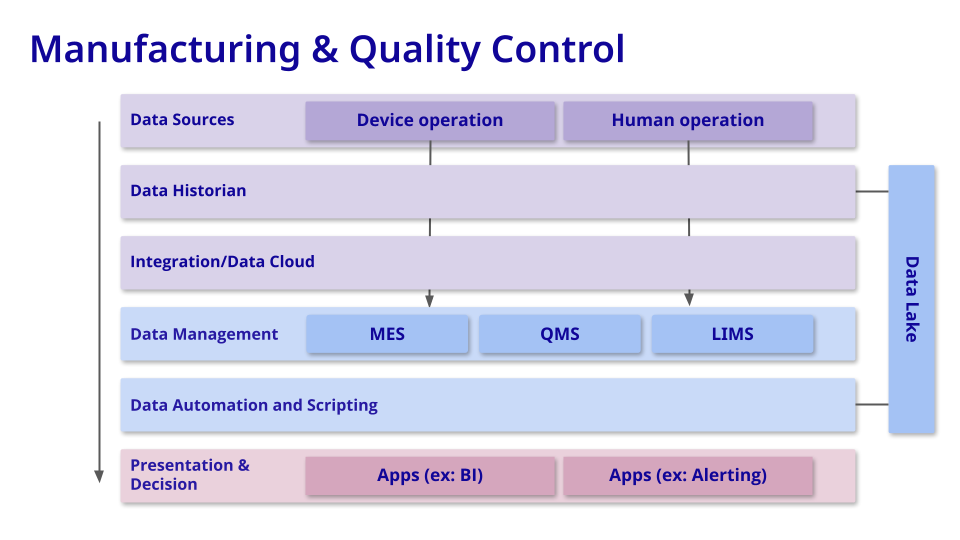
Measuring value: When assessing the value of software tools in the manufacturing and quality control stage of drug development, critical metrics include process parameter predictability, manufacturing downtime, and the count of data-preventable non-compliance events.
By tracking these metrics, teams can improve process reliability, minimize production interruptions, and reduce instances of non-compliance attributable to data-related issues.
Ongoing Assessment
Remember - digital development in the lab is a journey, not a destination
The successful navigation of each stage of biotech development demands a robust wet lab data tech stack tailored to address specific challenges and requirements. But consistency and continuity will be key. It’s the ongoing assessment of these metrics across phases that will paint a more holistic picture of whether technology investments are paying off, ultimately contributing to streamlined workflows and accelerated progress in drug development efforts.
By leveraging the right tools and technologies, biotech companies can unlock insights, drive efficiency, and ultimately accelerate the journey from discovery to commercialization, shaping the future of healthcare and life sciences.
We'll be expanding on this discussion in our next webinar with Merelogic's Jesse Johnson, where we'll also discuss the operation systems needed alongside these software tools to achieve a robust and powerful data foundation for your biotech.
Register below. Learn more at this link.
The insights gained from the webinar could shave years off your drug development pipelines, so you won't want to miss it. Make sure to register below.