Mobile Robots, Change Management, and More: Lab Automation Expert David Dambman Shares Insights on Successful Implementation
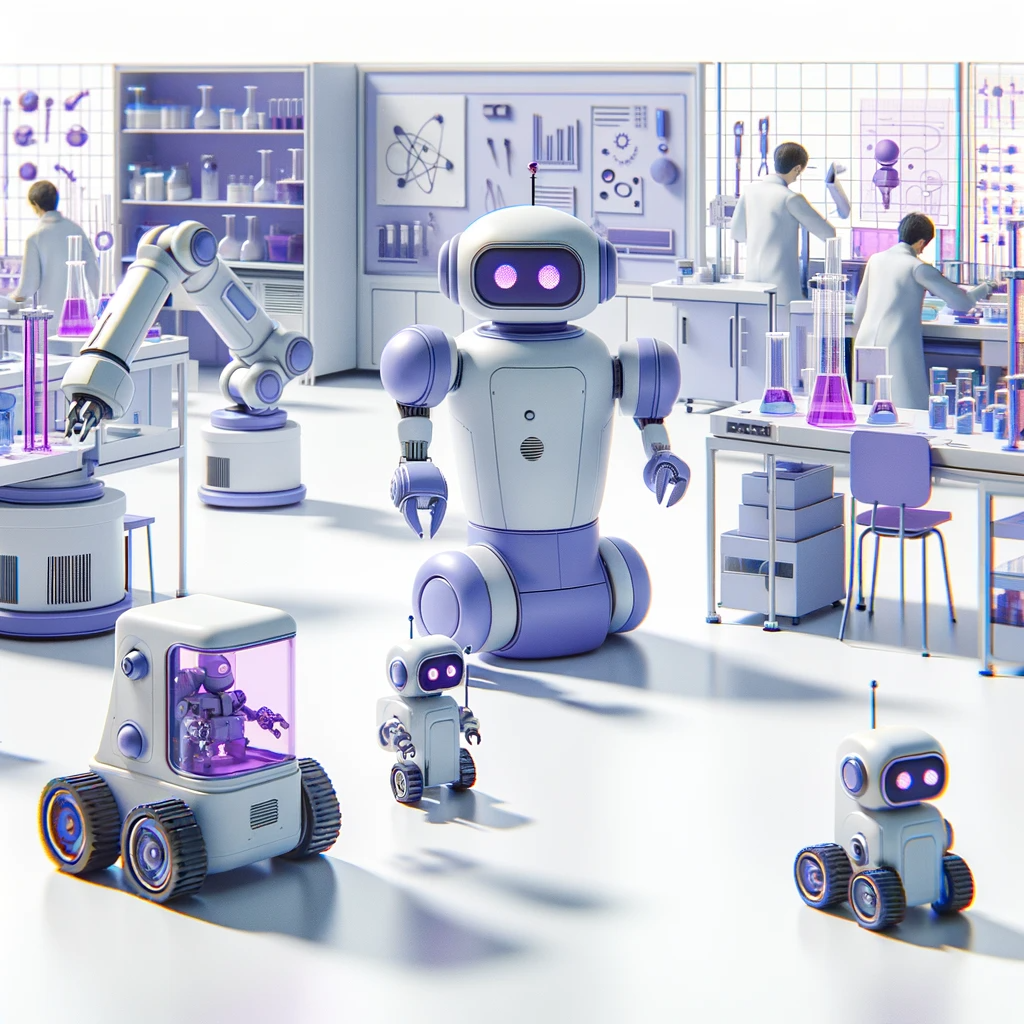
We’re excited to share the first post in our new Mission Logs series, a set of Q&A style blog posts with industry leaders, thinkers, and doers. Today, we’re showcasing a conversation with David Dambman, a true expert in automation, both in terms of robotics and data.
David is the former CTO of Biosero and Co-founder of LabSync, a lab automation consulting company and tech incubator. His thoughts on automation for the life sciences have been featured in talks at events including SLAS and publications such as BioIT World,Technology Networks, The San Diego Union Tribune, and more.
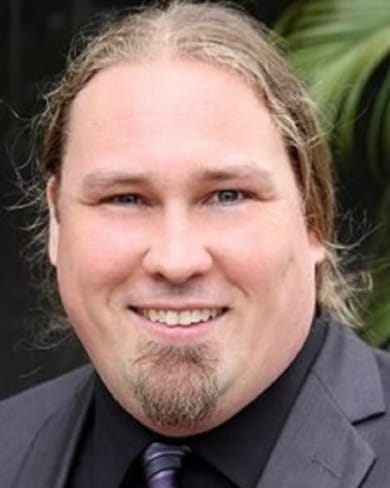
We sat down with David to pick his brain about:
- Why biotech falls behind other industries in automation
- Challenges that labs face in adopting automation
- Why automation projects fail
- How change management is a key part of success
- What he’s excited about most in automation
- And more
Read on for our interview with David—plus a fun fact about him, too.
Don’t Procrastinate: Now’s the Time to Automate
Q: At Ganymede, we often note how far behind the life sciences are when it comes to modern cloud-based infrastructure, data management, and automation. Why do you think that is? Are there challenges in adoption that are unique to the life sciences?
A: Actually building sophisticated automation takes a lot of capital investment, consensus-building, and a clear path to ROI—all of which take time to develop. With the life sciences, it’s often not well understood from the outside just how dynamic and highly innovative R&D is. By nature, that means that any infrastructure, automation, and standardization a lab builds will lag significantly behind the on-the-ground innovation.
Additionally, biology deals with a lot of unknowns. The models we’re working on are not as clear-cut as models in financial systems, for example. These inherent challenges based on the nature of the scientific domain makes it much more difficult to standardize and automate than normal in this field.
Q: Do you have any prime examples of where automation can be especially useful for labs, or where it’s a “no-brainer” to invest in? And how can companies identify those opportunities in their own labs?
A: The most classic use-case for automation is improving throughput without increasing headcount. It’s about looking at areas where there are high costs yet little ROI on those costs, like having a PhD-level scientist spending hours pipetting. Automation can leverage that individual’s work so they can be freed up for higher-level knowledge work.
Companies should look for an area where there’s a highly repetitive process—like pipetting— that takes up a lot of time. But it’s important to note that automation doesn’t have to be all-or-nothing. Not everything has to be automated right away. It’s good to take small steps, such as using an automated liquid handler to handle pipetting, or bringing in an automated stacker or a bulk dispenser.
I’m a big advocate of taking an evolutionary approach before trying to create an end-to-end system with no human intervention. Notch those small wins first.
Q: Speaking of starting small and building up: what are some of the most common barriers that labs face when translating human workflows into automation workflows?
A: Unlike a more traditional manufacturing model where a company might tool up and manufacture the same widget for five to ten years, pharma and biotech R&D are high-mix environments. There is a lot of adaptability and variability.
Humans are the most adaptable agents to perform work in a variable environment like this, because they can dynamically change what they’re doing to accommodate a constant data feedback cycle. So when it comes to automation, tools also need to be as adaptable to change alongside the process.
Labs need to think about not just a well-defined process to automate, but also how it will shift over time and as different variables come into play.
Q: Considering all the variability within labs, how do companies build in flexibility for nuances in process or unpredictable environmental factors—like fluctuating humidity—into an automated data flow or workflow?
A: There are so many things that humans do naturally that often are not captured in automation. For example, a scientist may subconsciously avoid exposing samples to intense sunlight through a window. This variable might not be tracked in a data system, and yet it could impact the results and reproducibility of the experiment.
Traceability is lacking for many of these sorts of processes, so building systems that can aggregate, associate and analyze data is a huge win. The unpredictable and dynamic nature of experiments often means that software is usually the best solution for doing this, not hardware. That’s why I usually advise companies to start with software first—Bits are easier to change than atoms. Start with an “integration-first” mentality. Connect your devices and manage the data coming out of them. For example, if you’re concerned about the variability in a particular assay, start monitoring the temperature, humidity, and other environmental factors.
Essentially, before introducing any automation elements, first understand what’s happening using a basic manual approach. Then labs can determine what processes, if guided by software with data connectivity, could ensure quality and give insights before investing further in physical automation.
Q: Are there other challenges or considerations that are commonly overlooked or underestimated?
A: There are more upstream and downstream considerations than people might think.
To automate, we need to introduce standardization to things like lab hardware, consumables, and the different data formats coming in and out of the lab. That data often flows into and out of other parts of the lab, teams, external partners, customers, and other places. Labs may be looking to solve one specific problem with automation—like throughput or walk away time—but they need to make sure those changes work within other groups and systems, too.
That’s why I advise people to break automation into three streams: the samples they’re processing, other consumables and materials, and data. Then look at all three and think about applying automation to one specific area—how does it impact the other categories?
Q: Automation isn't just about innovative technology: it requires a real culture change around how organizations view data and technology. How can companies start to shift their mindset around what automation is and what it can do for them?
A: When hardware and data automation projects fail, it’s not usually because of the technology itself. Sometimes it’s because the problems weren’t well understood, so the tech wasn’t properly fit-for-purpose. But more often than not it’s because there’s a failure to drive adoption within the lab. I’ve seen situations where the internal champion for automation left the company for another opportunity, but no one else really took to the technology. That’s a change management problem.
Labs can overcome this with a crawl/walk/run approach, bringing pieces gradually to show how effective automation can be. This approach can build trust and show how automation empowers people and improves their efficiency.
Q: Speaking of building trust, we know people can be wary of automation. How can labs overcome these perceptions to drive adoption?
A. With good training.
People can be afraid that automation is going to take their job, so it’s about showing people that automation isn’t a replacement—it’s an enabling tool. It gives them more time for knowledge work, new skill sets, and a way to 10x their productivity. For example, there’s a limit to how many wells an individual can pipette in a day, but one individual can manage multiple automated pipetting systems at once.
Generally speaking, people also want to understand and be in control of automation. I’ve seen employees who don’t want to “risk” their experiment with new technology. For example, if a scientist has just spent weeks preparing samples, they may want to do the next step by hand rather than risk a robot dropping the trays. They don’t want to start their automation journey on a big project, because that’s scary.
Organizations can partner with the company delivering their automation products, or appoint an internal team to make sure people know how to use the new tools and see their value. Again, a crawl/walk/run approach can be helpful here, too. Start by bringing automation into a simpler process, rather than on a big project. Help them develop skill sets and familiarity, and make sure they know how to recover from any problems that might arise.
Once your team has a baseline of how to use automation, then apply automation to more areas where the technology can bring the highest value.
Q: Which emerging technologies in lab automation are you most excited about?
A: At a high level, I’m most excited about the potential of mobile robotics, generative AI, cobotics, and the advancement of better UX/UI in lab software.
People are pushing back against older and harder to use tools and are expecting more. We’ve come to a point in technology where robotics can work alongside people in human space. From vehicles that can drive around the lab and deliver samples, to the ability to interact with natural language, we’ve lowered the barrier to interacting with robots and tech in the lab, because it can be done in a natural way.
Mobile robots have the power of shifting mindsets from a fixed equipment perspective—like a machine that stays in one place and is used 5 percent of the time—to a mobile mentality, where technology allows instruments to self-assemble into the right topology to meet a lab’s demands just in time. A mobile robot could bring equipment across the lab for a temporary job. It could bridge the gap between automation and instrumentation. These machines mean that, in the near future, we won't have to think anymore about building bespoke systems for one process—we’ll be able to think of a process and the system will build itself.
Full disclosure, I’m about to launch a mobile robot company, so of course I’m especially excited about this area of innovation!
Q: Where do you think there are opportunities for innovation that remain untapped?
A: We’ve seen a lot of growth in automation, but there are still more areas. As a field, automation cut its teeth on high-throughput screening: a single process done over and over again. Then automation grew and started to broaden to areas like next generation sequencing, so instead of testing millions of compounds against 1 target, you might have a 150-step process but are only running 4 plates a day. NGS is more complex, but it uses many of the same tools as high-throughput screening—liquid handlers, shakers, etc.—but with evolution on the software and data side.
The next, target-rich areas for innovation that I am really excited about are synthetic chemistry and cell culture. In synthetic chemistry, work is traditionally done by humans and software and hardware is often bespoke. So there’s a lot of need and room for off-the-shelf solutions. In cell culture, most scientists are still growing cells in flasks by hand. There’s innovation there already, but it’s slower than it needs to be with the ever-increasing demand. We need more data automation and tracking, especially.
Automation: An Area of Opportunity
A huge thank you to David for speaking with us about automation and sharing his wisdom. As companies face economic headwinds, David’s insights are more valuable than ever.
Companies will be asked to do more with their existing resources, and automation is an ideal way to increase productivity and give scientists back precious time, so they can focus on higher-value skills. Nobody wants their scientists doing “ELN days” or even “ELN weeks” when that time and energy could uncover the next big R&D opportunity.
So don’t procrastinate on getting your data and workflows in shape—start your automation journey today.
Join us at SLAS 2024 (Booth #685) in February or
Register for Our Upcoming Webinar with David
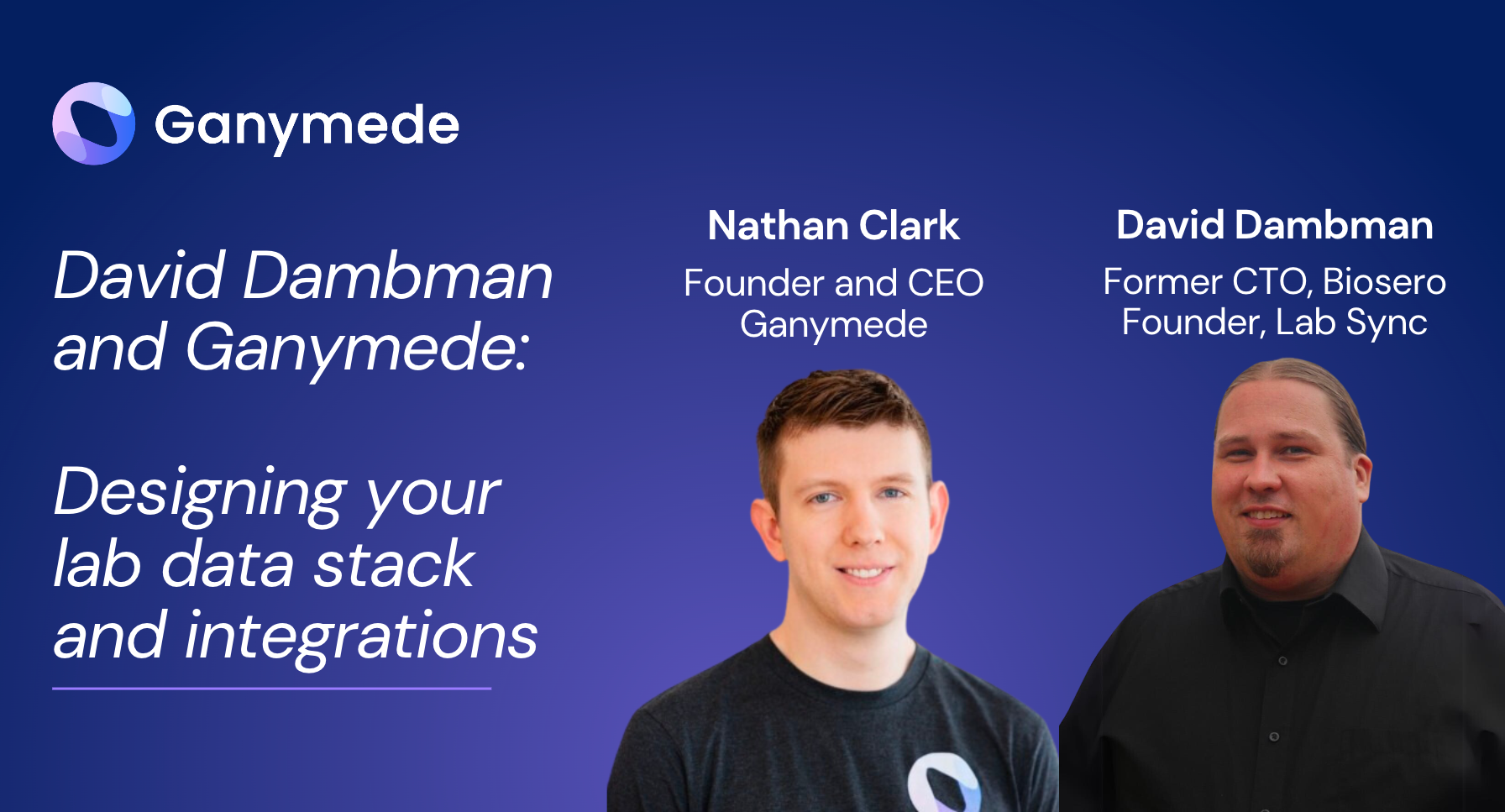