Four Data-First Strategies for Lab Automation
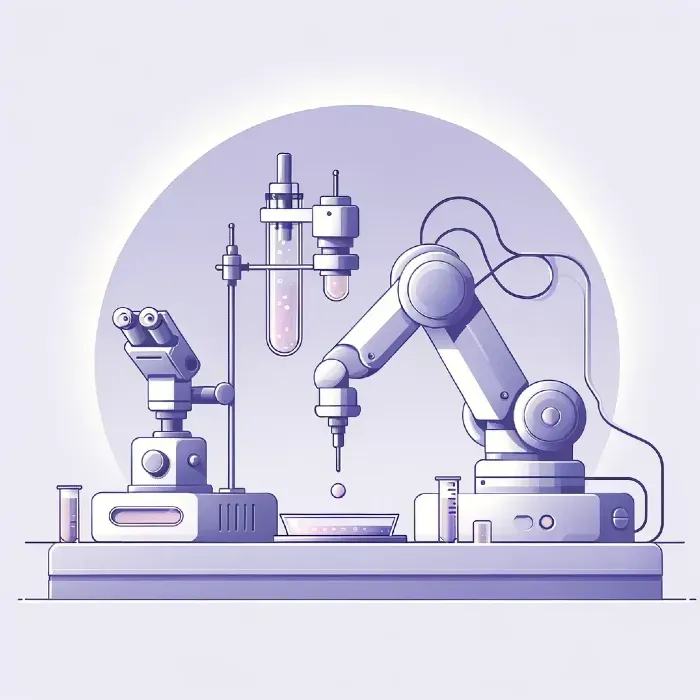
Imagine the lab of the future. Scientists are able to seamlessly conduct experiments across multiple instruments. With a few clicks, they generate amazing charts and uncover valuable insights. Everything flows smoothly and efficiently. And with that efficiency comes more time in scientists’ days. Time to think, discover, and collaborate.
That’s the future that lab automation offers biotech companies.
By automating work and data flows from all over the lab—including advanced instruments, apps like an electronic lab notebook (ELN) and laboratory information management system (LIMS), and even equipment like refrigerators—into the cloud, companies can realize many benefits. Some of the biggest boons of automation include:
- Putting time back in scientists’ hands for higher-value work (at Ganymede, we estimate as much as 20 percent!)
- Reducing errors in the data
- Speeding time to results
- And more
As exciting as these benefits are, automating a lab’s data can seem intimidating. After all, labs include dozens if not hundreds of data inputs, including from sophisticated instruments like liquid handlers and sequencers.
Starting your lab automation journey doesn't have to be scary. In a recent Q&A on our blog, automation expert and former Biosero CTO David Dambman gave some great advice:
“It’s important to note that automation doesn’t have to be all-or-nothing. Not everything has to be automated right away. It’s good to take small steps…I’m a big advocate of taking an evolutionary approach before trying to create an end-to-end system with no human intervention. Notch those small wins first.”
Understanding your lab’s data is a great way to get started with lab automation. If you’re looking for a few first steps, read on for four data-centric strategies.
1. Design an analysis before a lab automation system
Automation requires strong parameters and a clear goal. That’s why we always recommend starting an automation project with a well-designed and thought-out analysis. When designing your experiment, start by asking some key questions:
- What are the exact interfaces needed to collect the data?
- What datasets are necessary?
- What happens if my standards change?
Generally designing the data interfaces—such as precise input and output data structures—is a good way to build out that specific process. Whether you’re implementing a new lab instrument or a complicated liquid handler, you’ll then have a very precise definition of where data automation fits within the bigger process.
2. Shadow scientists for several days
The hand-made nature of science means there are many “unknown unknowns” when it comes to assay-generated data. Doing an experiment well is truly an artisanal process, and scientists are the ultimate craftspeople. They may not even realize all of the variables they introduce, such as minute differences in where they place samples. This sort of data is difficult to track, but it’s also crucial to ensuring repeatability and predictability.
Additionally, because conducting experiments is such a manual process in many labs, scientists spend huge amounts of time on tasks like walking USB keys between a piece of lab equipment and a PC to transfer data, moving files into an electronic lab notebook, and more. These are all prime examples of tasks that can be taken off scientists’ plates with data automation—thus freeing them up for more experiments, analysis, and other high-value work.
In short: Labs need to understand what scientists are doing both in the wet lab and on their computers to make sure every speck of data gets included in automation.
The easiest way to do this? Old school observation. Consider shadowing a few members of your team for a couple days to understand the small, manual steps that need to be taken into account for data automation. If they’re comfortable and willing, this could even look like strapping on a GoPro camera.
3. Make time for training and change management
There’s a saying in the business world: “Culture eats strategy for breakfast.” That’s true for automation too. Technology is just a tool. It’s ultimately dependent on the people that use it.
In order to succeed, a lab’s entire team needs to embrace lab automation and evolve their day-to-day processes to include it. Scientists need to know not just how to operate the tools day-to-day, but how to:
- Store data in the appropriate place
- Share data with others throughout the lab in a durable, findable way
- Store the full context of their experiment beyond just final, analyzed results
- Troubleshoot common issues and learn from instances of tech downtime (even the best automation systems have bugs!)
However, truly embracing automation goes beyond technical know-how. It’s a culture shift. People are often nervous about any new form of technology, and automation is no different. Without a bigger-picture context and vision for how automation can benefit the lab as a whole and their specific work, scientists can fear it will take away their jobs when, in fact, automation can enhance and boost their work.
This is why it’s crucial that labs provide technical training on any actual data-automation tools and build in time for change management. Ease your team into lab automation. Show them how the right tools and platforms take away some of their daily headaches. Bring things in gradually, so the team can see how much value lab automation brings to their day-to-day. (David Dambman has some great ideas on how to do this in our recent Q&A).
In short: before making a big push to automate, ensure your entire team is on-board and excited.
4. Push your cloud to its limit
Storing data in the cloud has never been cheaper or easier to do. An easy place to start with lab automation is by saving every speck of data you can. We mean it: everything!
Whether you have a private cloud set up in AWS or are using an off-the-shelf ELN, saving data is always worth it. Even if you haven’t automated anything data-related yet, having a trove of actual data to look at can help inform strategy and future decisions. And if there’s ever a question about the data for scientific review or long-term integrity, the information is findable.
Similarly, computing power is both cheap and robust. There’s been an explosion in recent years of cloud-native tools and platforms like Ganymede designed specifically for the life sciences. In parallel to saving all your data, explore more advanced solutions. Learn how serverless platforms work and the benefits they bring to your team. Think about the right lab automation tech stack for your organization (or tech sandwich, as we like to think of it).
Get Started with Lab Automation Today
By automating work and data flows, biotech organizations are taking one big step toward building a lab of the future. From saving scientists’ time on tedious work, to making data FAIR, to speeding up discoveries, well-managed data brings many benefits to labs. If lab automation is on your roadmap for this year, the four strategies above will set you on a strong path.
Join us at SLAS 2024 (Booth #685) to Learn More About Automating Your Lab Or Watch our On-Demand Webinar About Lab Integrations
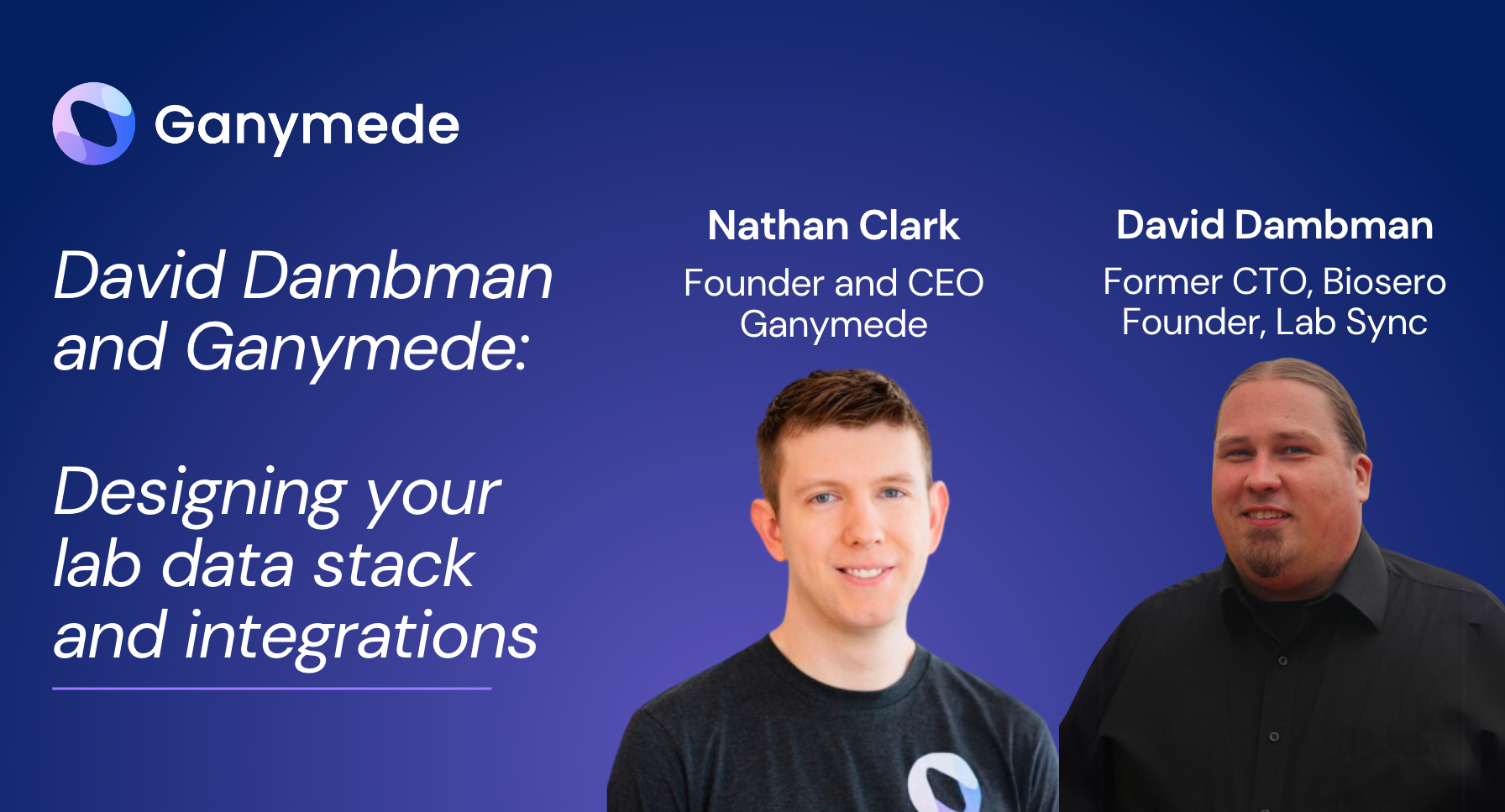