How Not To Get Overwhelmed By “Data Strategy”: Expert Jesse Johnson Shares What it Takes to Succeed with Scientific Data Management
Scientific data management is a crucial part of any lab’s success. Jesse Johnson, author of the Scaling Biotech newsletter, shares insights on how to get data in shape.
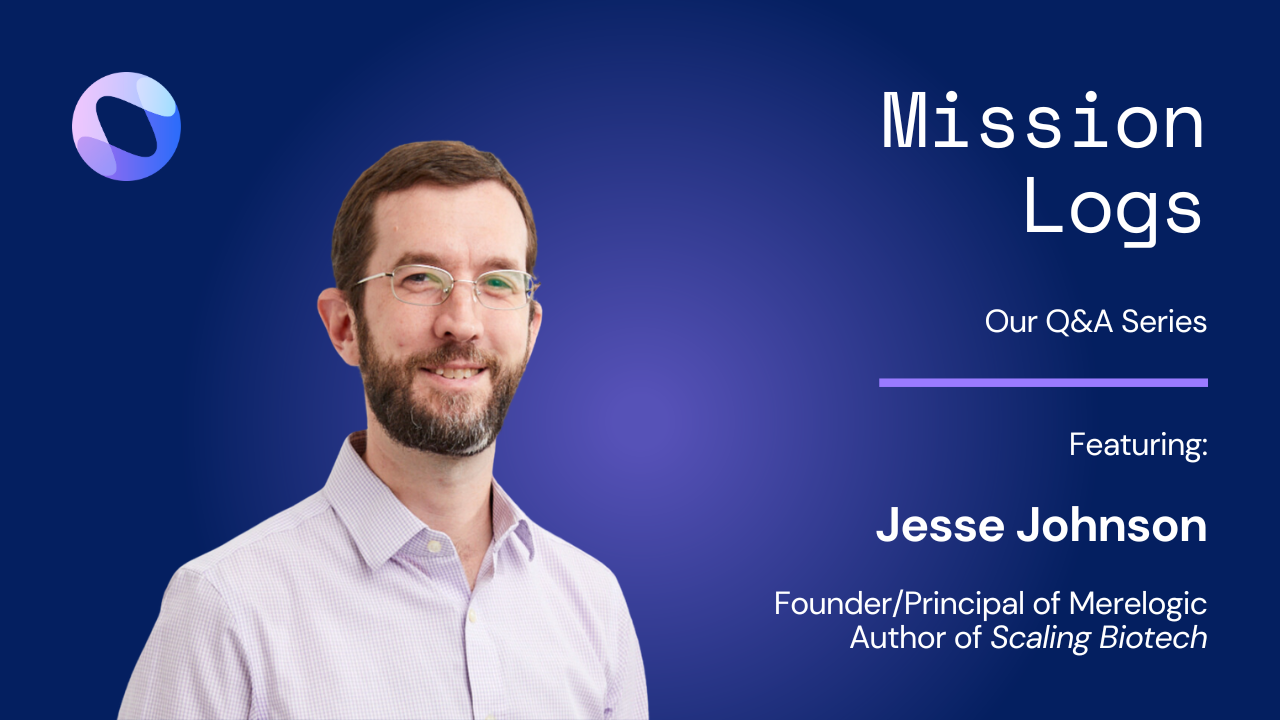
Last month, we launched our new Mission Logs series of blog posts, where we interview leading thinkers at the intersection of data and biotech. After kicking things off with a focus on wet lab automation, we’re thrilled to tackle the topic of scientific data management—why it matters, where it goes wrong, and how labs can wrap their arms around it.
Today, we’re joined by Jesse Johnson, a leader and expert in lab data management.
A former researcher and professor, Jesse has extensive experience with life science data and software engineering at companies including Verily, Sanofi, and Cellarity. He now consults with biotech companies on their scientific data management strategies as the Founder and Principal of Merelogic. His weekly newsletter, Scaling Biotech, is a treasure trove of insights into biotech data management practices.
I recently spoke with Jesse about how life science companies can shift their thinking around data, including:
- Why “data strategy” can be the wrong way to think about wrangling data
- The importance of mundane data management and engineering principles
- Why ELNs aren’t enough for data science, AI, and other advanced uses of wet lab data
- How metadata falls off the radar for companies
- And more.
Keep reading for my Q&A style interview with Jesse.
Data Strategy Isn’t the Right Term for Scientific Data Management
Q: We’ve heard a lot lately about AI in biotech. But as exciting as advanced tools like AI may be to biotech companies, we all know those tools only work as well as the data underpinning them. Data strategy is an important part of getting ready for advanced technologies—what does it take to create a really great one?
A: I actually don’t like to use terms like “data strategy.” People tend to get overwhelmed by the concept. It sounds like speaking with a large, expensive consulting firm for multiple months. Sometimes companies go that route, spending lots of time on planning that never comes to anything. Or, for startups, they may think they’re too early to get started. In both cases, the organizations lose time—and data problems keep piling up, creating a huge backlog to clean up.
It’s better to find some middle ground between an overwhelming data strategy and doing nothing. Organizations can make small shifts, one step at a time. I like to think of the process as an evolution.
For example, when it comes to organizing data, companies can start by simply adopting a consistent folder structure within a cloud-based, data science-ready system like AWS S3 or Google Cloud buckets. From there, they can gradually layer on software and automation, like Ganymede, that make data collection, storage, and management more consistent and with minimal attention from users.
Q: That sounds almost like going back to basics: focusing on the underlying infrastructure and data management 101, rather than on the advanced tools that get layered on top—the “mundane” stuff, as you called it in one of your recent Substack posts. What are the sorts of “mundane” things companies should focus on when trying to get their lab data in shape?
A: There’s a pattern in software and IT where people do work they feel is important, but it goes unseen. It’s not glamorous work, and people only recognize its importance and value when it breaks. These are things that aren’t considered directly connected to science itself, because it’s not work that’s looking at specific cells, proteins, etc. However, if that data and IT work doesn't happen, the science can’t happen either.
One example is getting the entire lab to agree on where to put data. Too often, labs will store data all over the place. Then, in three to six months, the only way to find information is to ask someone. If that person is no longer at the company, you’re in trouble. There are advanced data storage solutions to solving this issue, but even something as simple as agreeing to put data into SharePoint or Google Drive is an improvement on storing it all over the place. Organizations can create a document that lays out a directory structure and get everyone to agree to it. If they don’t organize the data, that starts to get in the way of the science.
So in my view, focusing on the “mundane” is trying to reclaim that dynamic—to recognize it’s important work to be proud of.
Q: Many of the labs we speak to use their ELN to capture everything in lieu of a dedicated cloud storage solution. How do you think about ELNs in the context of managing scientific data?
A: The biotech industry is in a transition phase right now with ELNs. In fact, I’m almost ready to predict the death of ELNs! If you look at the historical reasons ELNs were adopted, it was driven by the US patent system as a way to document invention timelines. Since the patent system was based on when something was discovered (first to discover), companies needed to prove they’d found whatever molecule, insight, etc. first. The ELN was essentially a tool for lawyers to prove discovery.
However, in 2013, the USPTO changed the patent system to be first to file. This essentially removed one of the original purposes of ELNs. Today, one of the major driving factors for recording experiment data digitally is data science, computational biology, AI/ML, etc. ELNs are not ideally set up for these uses. Data science teams in computational biology need data in a structured format, which is something traditional ELNs don’t have, or have as an add-on that doesn’t work very well. However, ELNs are still used because scientists have built the habit of entering data there.
Of course, there are also LIMS platforms that capture structured data. However, these are mostly used in tightly controlled and repeatable lab processes that don't work in early discovery.
So companies need something between an ELN and LIMS product. They require something with the structure of LIMS to extract data, but that’s also flexible enough to support early stage discovery, where companies may run an assay once or a few dozen times.
Q: For companies trying to implement the so-called mundane steps to improve their scientific data management with modern infrastructure solutions and practices, what’s the best place to begin?
A: I like to start from the perspective of real-life processes or decisions that need to be supported, and then work backwards to how we support those things. Life science companies constantly need to make a series of decisions, such as selecting an indication, targets, hits and leads, when to start the IND process, and more.
The best way to make each decision of these decisions is to use the right data. That means being intentional about designing and planning experiments, generating the data, analyzing it, and, finally, making the decision. If companies look at each stage, they can break it down more to what’s necessary to accomplish each step. Then it’s possible to ask questions to inform the management approach, like: how consistent does this need to be? How much infrastructure and process do we need, versus how much of this can be done ad hoc?
Q: Are there any common pitfalls you see when it comes to data management, even with software in place?
A: One of the biggest pitfalls I see has to do with metadata. Organizations are so focused on the data, they forget about the metadata. To distinguish the difference, data is anything that comes out of an instrument, such as a plate reader, sequencer, digital microscope, etc. Metadata is keeping track of everything that happened before the instrument—what was added into the plate, what the scientist did to cells, a description of how that sample was prepared, etc.
If labs aren’t tracking metadata, they’re losing valuable context. If you run a plate through an instrument, it gives you a reading from well A3 and a different reading from B17. But if you don't know what was in each well—what kinds of cells, how they were treated, etc.—there's no way to interpret those readings.
Unfortunately, the software to actually manage metadata the way data scientists need it is still in its infancy. I hope to see more companies bridge that gap and provide tools to capture metadata in a more consistently structured form.
Q: Beyond the tech itself, how can companies working on digital transformations ensure success around all the non-tech elements, like people, processes, etc?
A: Change management is a very complex subject. In my experience, the vast majority of bench scientists are totally on board in principle. Oftentimes, the bottleneck isn’t the bench scientists being willing—it’s that downstream data scientists don't know what to ask the scientists to do upstream. They know something is wrong, but not how to fix it. Additionally, bench scientists have a lot to do, so even if lab leadership brings in new software, at some point the scientists get slammed and do what’s familiar. In short, it’s not about convincing scientists that new software and data management tools have value, it’s about making the habits stick.
Practically speaking, I often recommend starting with a mechanical turk approach to help with this. Think of what any given piece of software or code needs to do, and have the team start by doing it manually—such as gathering data in a consistent template, or moving data by hand into a specific place. Then build software around the process. You’ve essentially created a space where software can be added. This will be more work for scientists in the short term until you implement the software that automates those tasks, but this process provides much more flexibility, ensures the right fit, and builds appreciation for the final product.
Ultimately, I think a lot of success comes down to making data management consistent AND making processes easier. But if labs can’t do both, the most important thing is not to make things harder.
Scientific Data Management: The Foundation for AI, ML, and Other Advanced Tech
Thank you to Jesse for sharing his insights with us today.
Scientific data management may seem like a dry term, but it’s clear from speaking with Jesse just how fundamental it is for labs to succeed. As he pointed out, labs are driven today to collect data not just for IP reasons, but also for reasons of computational biology and complex algorithms. This changes what labs need from software—and it changes how sophisticated they need to be about data collection, storage, and management.
Without a good understanding of scientific data, labs today will fall behind their competitors. That’s why it’s more important than ever for life science organizations to approach scientific data management strategically—even if they don’t have a “data strategy.”