The Ins and Outs of Lab Automation in 2025
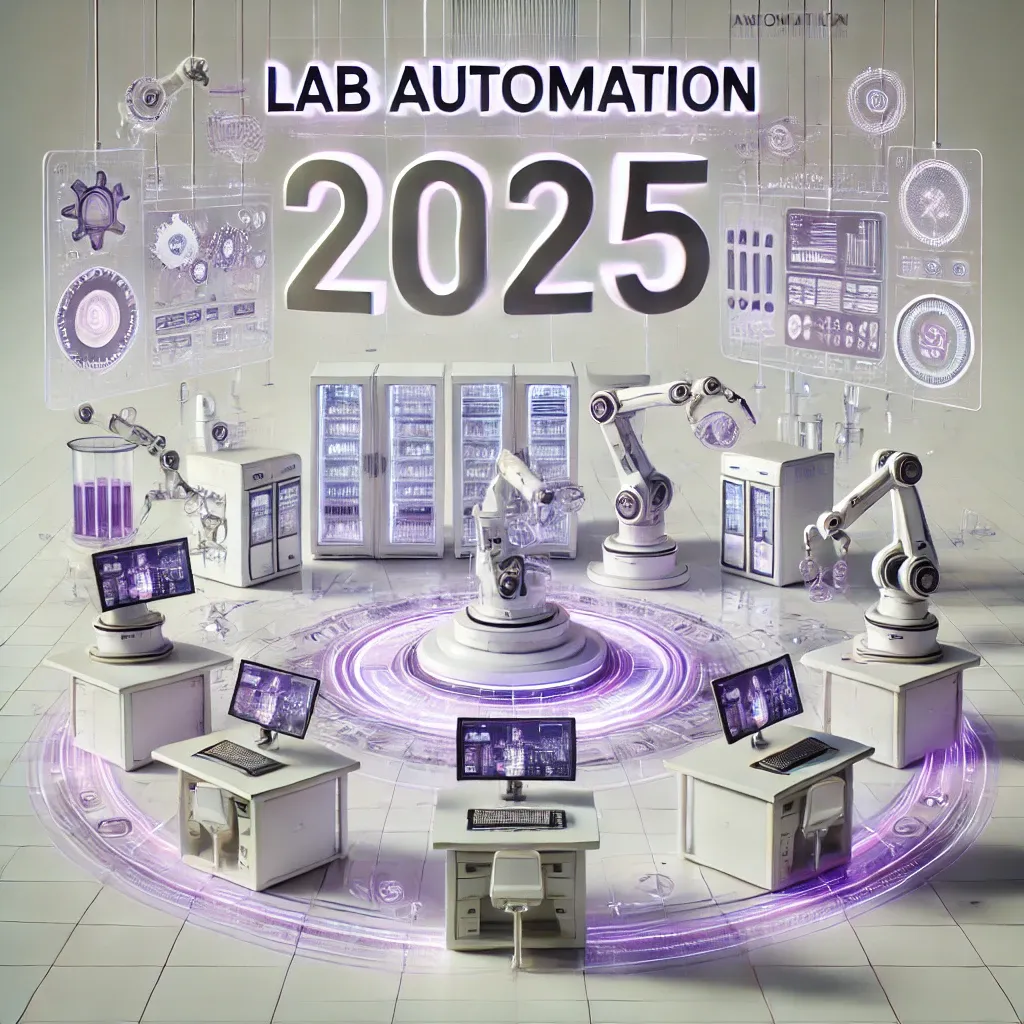
In #1: Modular Data and Lab Systems - For years, labs have been racing to build out advanced workcells—purchasing shiny, custom hardware solutions to automate processes. The result has often been a collection of “islands of automation,” each with its own protocols and data silos. Now in 2025, the frontier lies in weaving these islands together with modular software systems that plug and play with any piece of lab equipment. The shift is inspired by decades of learning in software engineering: from microservices that let developers build resilient applications, to well-defined APIs that ensure data can move freely between modules. By applying these abstractions to the lab, scientists can automate entire workflows seamlessly, reduce friction in data exchange, and accelerate discovery. This is less about building bigger robots and more about creating universal data “connectors” that treat labs as integrated, flexible systems—unleashing the true potential of the instruments you’ve already invested in.
In #2: Magnetic Levitation Decks for Workcells and Mobile Robotics - A new wave of motion control has hit the lab floor: magnetic levitation decks and vehicles that glide from station to station without mechanical rails. These systems rely on contactless magnetic fields to move labware, reagents, or even entire sub-assemblies between instrumentation. Without the need for physical connections—tracks, cables, or gear-driven arms—labs can achieve cleaner, higher-throughput workflows and dramatically reduce maintenance downtimes due to mechanical failures. Equally important, magnetically levitated robotics can dynamically reroute paths in response to shifting priorities. This allows labs to sidestep bottlenecks and manage instrument usage more flexibly, almost like local traffic control for lab assets. Keep an eye out for how these “floating” platforms become mainstream, pushing hardware reliability to new levels and letting lab managers reorganize workflows on the fly.
In #3: Copilots over Generic Generative AI - Large language models (LLMs) caused plenty of buzz when they first arrived, but many R&D teams found them ill-suited for generating domain-specific insights or guaranteeing scientific correctness. Instead, the real utility in 2025 comes from “copilots”: specialized AI assistants that understand a narrower scope, such as experiment design or lab software configuration. These AI copilots help scientists encode complex processes into protocols, guide them through setting up automation tasks, and even generate the syntax for specialized tools—all while leaving the scientific reasoning to the experts. By focusing on the “scaffolding” around research rather than the scientific breakthroughs themselves, these copilots strike a balance: accelerating daily tasks without risking misinformation or unvalidated outcomes. Expect to see AI copilots built directly into lab management software, instrument control interfaces, and data analysis pipelines.
In #4: Scientists Who Can Code - Gone are the days when “techbio” simply meant pairing non-technical lab researchers with software engineering teams. In 2025, a new breed of scientist is emerging—one who can both design experiments and write Python scripts right at the bench. With modular tools, robust APIs, and user-friendly programming libraries, more scientific workflows can be automated by the very people doing the research. This closer coupling of bench work and code shortens the feedback loop from hypothesis to data to refinement. Rather than waiting for a dedicated engineering queue, scientists can script custom workflows, process data in real time, and tweak assays on the spot. The result is a more dynamic lab environment—one where the scientist-coder becomes a pivotal force in bridging experimental ideas and digital infrastructure.
Out #1: Microfluidics - A few years ago, microfluidics was heralded as a disruptive technology that would miniaturize entire labs onto chips. But despite undeniable advances in certain niche diagnostics, the broader promise of a universal “lab-on-a-chip” never quite panned out at large scale. High development costs, reliability challenges, and frequent design iterations made microfluidic setups too cumbersome for broad adoption, especially in big pharma workflows that rely on established instruments. While microfluidics has found its place in specialized assays—like single-cell analysis or point-of-care diagnostics—it no longer headlines the automation conversation the way it did in the late 2010s.
Out #2: Departmental Software Licensing - The Software-as-a-Service explosion of the past decade left many large pharmas with a patchwork of departmental licenses—think 20 different ELNs, LIMS, and analytics platforms scattered across R&D. But with budgets tightening and data fragmentation hampering cross-team collaboration, 2025 sees central IT teams reining in the chaos. It’s no longer efficient (or even feasible) to manage dozens of overlapping systems. Instead, organizations are converging on more unified platforms, enabling consistent data models, streamlined governance, and easier vendor management. While specialized solutions still exist, the trend is towards consolidating and integrating them via robust APIs—rather than letting each department spin up its own standalone software in a silo.
Out #3: Legacy Scientific Data Visualization Tools - Tools like JMP, Spotfire, and GraphPad once dominated scientific graphing and analysis, but their old-school interfaces and limited adaptability are starting to show their age. Over the past year or two, a surge of data visualization startups—many born out of the COVID-driven wave of bioinformatics innovation—have begun to break these legacy strongholds. The new tools offer slicker user experiences, real-time collaboration, and big-data readiness, allowing teams to handle everything from raw instrument signals to multi-omics data. Many also integrate seamlessly with laboratory automation platforms, letting users visualize data in context rather than exporting to separate apps. While the classic tools won’t vanish overnight, the momentum is clearly shifting to fresh entrants that combine modern UX with the flexibility and scale that life science research now demands.