From Indication to IND: Building Your Lab Data Tech Stack
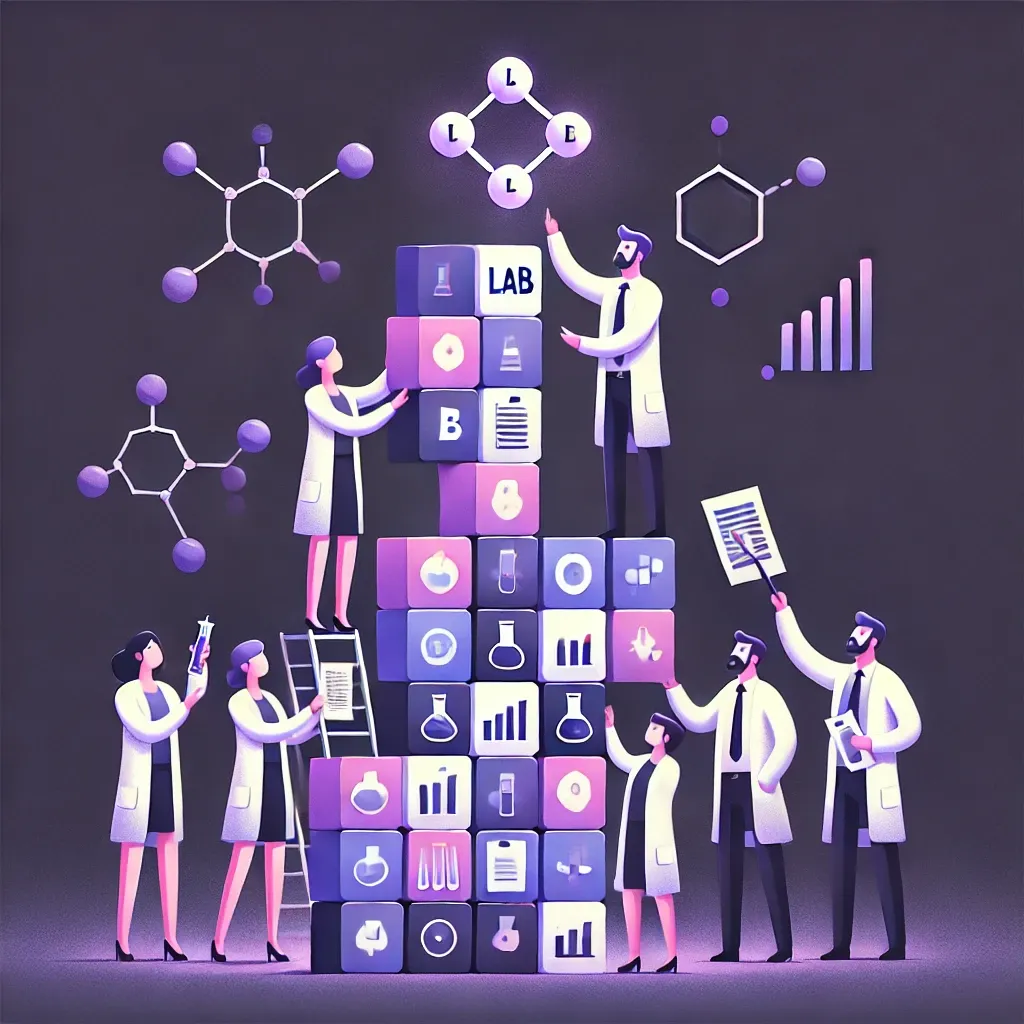
Labs need a sophisticated data stack. In fact, an advanced lab data tech stack is just as important as advanced scientific equipment.
Modern lab data tech stacks should help labs answer key questions about their data, including:
- What did a person do, or what should they do? To understand human interfaces with data, a modern data stack will have human data entry and process tools, such as ELNs, manufacturing execution systems (MES) / laboratory execution systems (LES) in high-scale contexts like manufacturing, or electronic data capture (EDC) systems for trials
- Where does the data go? A scientific data management system (SDMS) or other type of instrument data capture and management system helps determine where data flows.
- Where do the metadata and outcomes go? Because metadata is just as important as raw data, it, too, must be tracked with structured data systems of record such as molecule registries, LIMS, inventory or instrument management systems, and/or a clinical trial management system (CTMS) in the case of organizations running clinical trials.
- How do you use the data? Further downstream, how end-users actually utilize data adds tools to the tech stack. Data visualization, analysis, and pipelining/workflow automation systems are key.
- How do you control the process? Last but not least, quality becomes a big consideration as companies mature. That’s why quality and document management systems such as quality management systems (QMS) come into play.
A thoughtful, well-integrated data stack enables labs to gather, organize, track, and analyze data like never before. Data becomes FAIR: findable, accessible, interoperable, and reusable.
With well-organized, FAIR data, a great data stack also allows labs to invest in lab data automation to complement efforts on the equipment side of lab automation and to prepare for advanced AI tools. This also all helps with traceability and quality factors that ensure the reliability of your data, and make finding data faster when debugging experiments.
The Essentials of the Modern Lab Data Tech Stack
Once data is generated by one of these many sources, it flows through a series of layers along its journey that we use to segment our recommendations by stage:
1. Data Capture and Integration: The point at which data flows off instruments, apps, third-party sources (like CDMOs or other external partners) into a centralized location, where it is standardized, cleaned, and made ready for use.
2. Data Management and Analysis: Once data has been captured, labs need to keep it somewhere. This layer comprises tools and best practices to store, clean, and analyze data intelligently.
3. Data Automation and Planning: By capturing lots of data and managing it well, labs can begin to automate the flow of that data from inception on lab instruments through to an ELN and a scientist’s final analysis—and back again.
4. Presentation and Decision Making: At this final stage, all that FAIR data makes it into the hands of the non-technical end users—typically scientists—for entry into applications for analysis.
There are many sources of data within organizations, such as analytical lab instruments, equipment like fridges and their temperatures, third-party contract design and manufacturing organizations (CDMOs) or other partners, and more. Each of these stages in the data’s lifecycle, from origination through advanced analysis, requires a thoughtful suite of SaaS or enterprise software tools, platforms, and solutions.
However, data is more complex than just its lifecycle. The company’s overall position in the drug development lifecycle matters, too.
For example, an organization manufacturing an FDA-approved product at scale around the world has very different needs than an early-stage biotech doing target ID in one geographical location, like Boston or San Francisco. Thus, considering the company’s stage of development is equally crucial, because each stage will have its own priorities and needs for how data is handled and managed.
Therefore: the best software for each layer of the data tech stack will also matrix against each organization’s specific stage of development. Based on that we've segmented the recommendations of key tools you need to consider by each stage into the following image below.
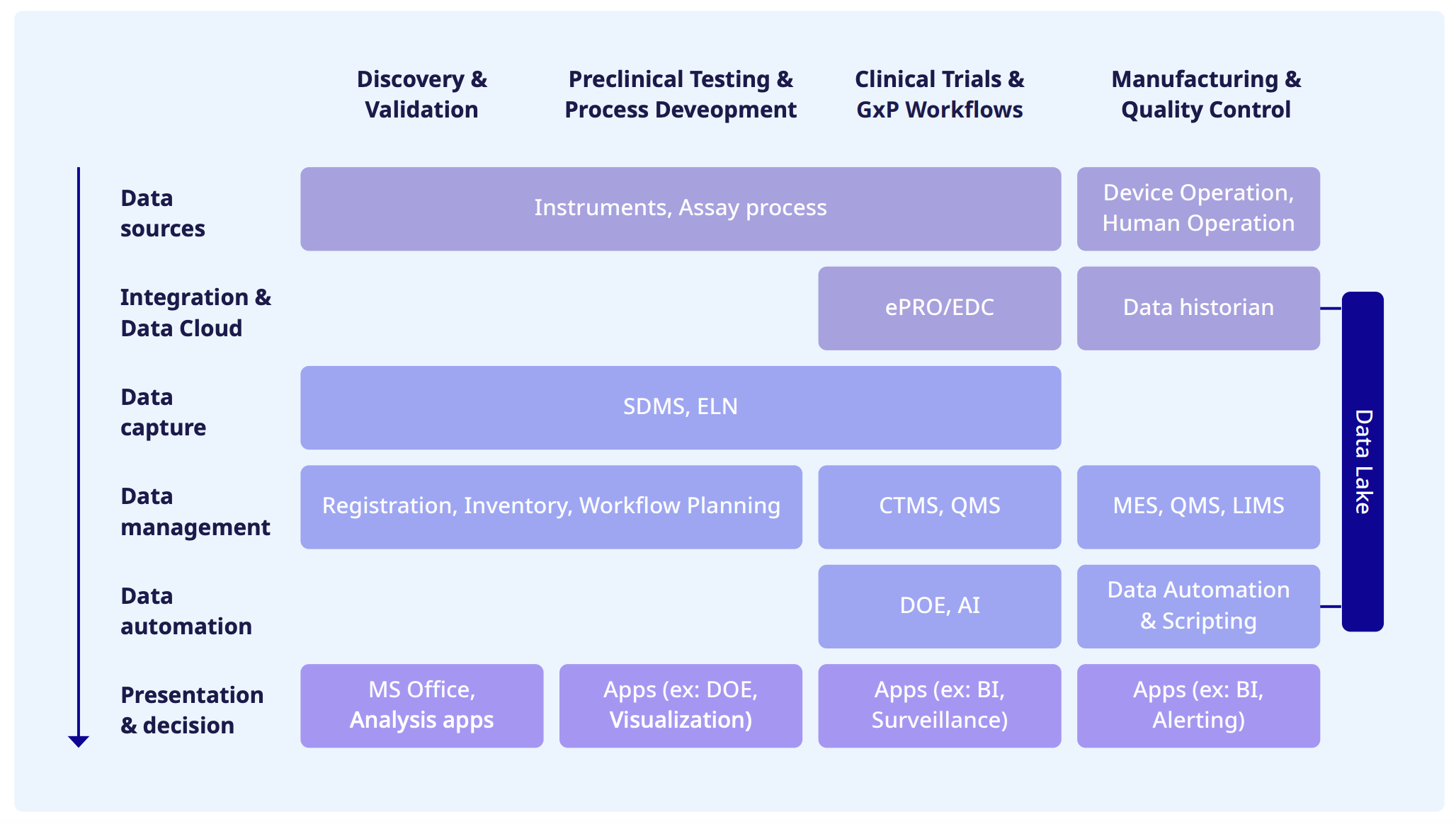
Click here to download the full 16-page ebook to see our technology recommendations and evaluation framework in-depth for each stage, along with key "dos and don'ts" across technologies.
Questions or want to learn more? Contact us here or reach out any time to hello@ganymede.bio with questions!