6 Questions with Benson Lee, our Co-Founder & Head of Implementations Benson Lee
A new installment in our "Meet the Team" series.
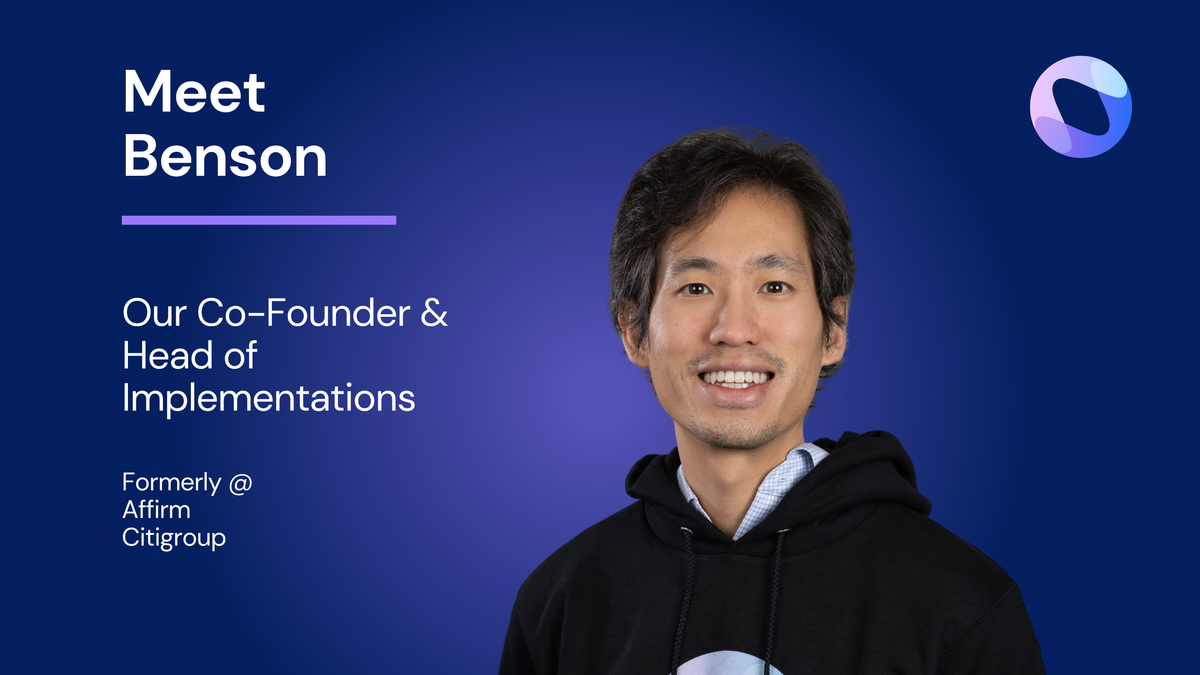
Name: Benson Lee
Title: Co-Founder & Head of Implementations
Office: Bay Area
Inspired by his experiences working in structured finance and wanting to make a meaningful contribution to society, our Co-Founder Benson Lee decided to "trade" in his role for a new one, instead building structured data solutions for the life sciences industry. Now that he's returned to his roots in biology, Benson spends his days enabling scientists with data superpowers as the leader of our implementations team. Get to know him better in our interview below, and learn what insights he was to share with entrepreneurs in biotech:
1) Could you tell us a little bit about your background? Where were you before you co-founded Ganymede?
Prior to Ganymede, I worked for 15 years in structured finance, most recently running Quantitative Markets at Affirm. The group was responsible for building ML models and structuring asset-backed securities - so ostensibly using data for very different applications. The common thread shared by both finance and biology is that both domains involve large quantities of data in complex systems, where partial understanding is the rule rather than the exception.
In structured finance, some of the core technical challenges involve establishing operating parameters to efficiently price and transfer risk. In biotech, this plays out as folks are testing samples - for which the conceptual understanding is rooted in biology and chemistry - with instrumentation containing many other knobs - rooted in physics and engineering.
How complete of a portrait does your data present? How quickly do you have to translate your data into decisions? What is the proper balance between precision and effort for extracting data value? The characteristics of the questions to answer determine the appropriate team compositions and expertise to address them.
2) What was your inspiration for co-founding the company? Any wisdom you’d want to share with those trying to launch their own start-up?
My main goal in starting Ganymede was to find an effective way to channel my work into something that I thought would benefit society most. In my case, I had spent some time doing plasmid construction in a basic research lab, and had always had an interest in domains with sophisticated tooling and partial knowledge. Doing BLAST searches to reconstruct the likely sequence of a protein led me towards studying computer science many years ago, so - in some ways - I’m just going full circle here.
My main advice for individuals considering a startup would be to consider what makes you uniquely qualified for something; everyone collectively acquires experience down paths that look nothing alike upon close inspection. There are a tremendous number of worthwhile things to do and no reason for everyone to work on the same problems; in short, you do you.
3) We’re coming up on Ganymede’s 2-year anniversary. How would you describe the growth and development of the company so far? Any favorite memories?
The company has come a long way from the early days of two folks spending their nights building a website to tell their story to one with some organizational structure, albeit one where each team is still under the two pizza box threshold. My favorite memories have been around seeing and collaborating across the company as everyone puts their pieces into the broader puzzle we’re addressing.
As products are built, I’ve loved watching how generous and willing teams are to share what they’ve built and how open and receptive they are to feedback from different perspectives.
4) Looking at 2024 and beyond, which technological or scientific innovations are you most excited about?
From a modeling perspective, I’m most excited about the progress that has been made in recent years on developing deep learning theory, which has been foundational for dramatically reducing the amount of trial-and-error that enters into building machine learning models. It’s been incredibly exciting to see how much popular interest and capital investment has increased in these applications.
Beyond natural language applications, I’m looking forward to seeing this innovation permeate to different areas of science and engineering, and to seeing how much we can get future scientists to operate to the top of their license.
5) What’s one common misconception, misunderstanding, or misused technologies that you’d love to resolve?
One common misconception regarding implementations is that technical challenges are the primary ones for a successful implementation. The thornier problems tend to involve coordinating efforts across multiple groups that may not directly interact. How can processes be designed that conform well with existing practices held by different teams? Who informs data strategy and governance - and how are these aspects maintained? An implementation that doesn’t address these questions from inception stands a good chance of being a solution tackling the wrong problem.
6) If you could give one piece of advice to anyone looking to break into this space at the intersection of biotech and tech, what would it be?
Reach out! The group of individuals working in this intersection is small, fervent, and open - which makes it ideal for anyone interested to join the community. There are more ways to contribute than any one person can be aware of, so having many conversations will give you the best chance at finding where you fit best.